mirror of
https://github.com/comfyanonymous/ComfyUI.git
synced 2025-01-11 18:35:17 +00:00
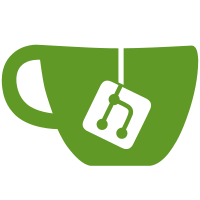
Use a simple CLIP model implementation instead of the one from transformers. This will allow some interesting things that would too hackish to implement using the transformers implementation.
774 lines
27 KiB
Python
774 lines
27 KiB
Python
from inspect import isfunction
|
|
import math
|
|
import torch
|
|
import torch.nn.functional as F
|
|
from torch import nn, einsum
|
|
from einops import rearrange, repeat
|
|
from typing import Optional, Any
|
|
from functools import partial
|
|
|
|
|
|
from .diffusionmodules.util import checkpoint, AlphaBlender, timestep_embedding
|
|
from .sub_quadratic_attention import efficient_dot_product_attention
|
|
|
|
from comfy import model_management
|
|
|
|
if model_management.xformers_enabled():
|
|
import xformers
|
|
import xformers.ops
|
|
|
|
from comfy.cli_args import args
|
|
import comfy.ops
|
|
|
|
# CrossAttn precision handling
|
|
if args.dont_upcast_attention:
|
|
print("disabling upcasting of attention")
|
|
_ATTN_PRECISION = "fp16"
|
|
else:
|
|
_ATTN_PRECISION = "fp32"
|
|
|
|
|
|
def exists(val):
|
|
return val is not None
|
|
|
|
|
|
def uniq(arr):
|
|
return{el: True for el in arr}.keys()
|
|
|
|
|
|
def default(val, d):
|
|
if exists(val):
|
|
return val
|
|
return d
|
|
|
|
|
|
def max_neg_value(t):
|
|
return -torch.finfo(t.dtype).max
|
|
|
|
|
|
def init_(tensor):
|
|
dim = tensor.shape[-1]
|
|
std = 1 / math.sqrt(dim)
|
|
tensor.uniform_(-std, std)
|
|
return tensor
|
|
|
|
|
|
# feedforward
|
|
class GEGLU(nn.Module):
|
|
def __init__(self, dim_in, dim_out, dtype=None, device=None, operations=comfy.ops):
|
|
super().__init__()
|
|
self.proj = operations.Linear(dim_in, dim_out * 2, dtype=dtype, device=device)
|
|
|
|
def forward(self, x):
|
|
x, gate = self.proj(x).chunk(2, dim=-1)
|
|
return x * F.gelu(gate)
|
|
|
|
|
|
class FeedForward(nn.Module):
|
|
def __init__(self, dim, dim_out=None, mult=4, glu=False, dropout=0., dtype=None, device=None, operations=comfy.ops):
|
|
super().__init__()
|
|
inner_dim = int(dim * mult)
|
|
dim_out = default(dim_out, dim)
|
|
project_in = nn.Sequential(
|
|
operations.Linear(dim, inner_dim, dtype=dtype, device=device),
|
|
nn.GELU()
|
|
) if not glu else GEGLU(dim, inner_dim, dtype=dtype, device=device, operations=operations)
|
|
|
|
self.net = nn.Sequential(
|
|
project_in,
|
|
nn.Dropout(dropout),
|
|
operations.Linear(inner_dim, dim_out, dtype=dtype, device=device)
|
|
)
|
|
|
|
def forward(self, x):
|
|
return self.net(x)
|
|
|
|
def Normalize(in_channels, dtype=None, device=None):
|
|
return torch.nn.GroupNorm(num_groups=32, num_channels=in_channels, eps=1e-6, affine=True, dtype=dtype, device=device)
|
|
|
|
def attention_basic(q, k, v, heads, mask=None):
|
|
b, _, dim_head = q.shape
|
|
dim_head //= heads
|
|
scale = dim_head ** -0.5
|
|
|
|
h = heads
|
|
q, k, v = map(
|
|
lambda t: t.unsqueeze(3)
|
|
.reshape(b, -1, heads, dim_head)
|
|
.permute(0, 2, 1, 3)
|
|
.reshape(b * heads, -1, dim_head)
|
|
.contiguous(),
|
|
(q, k, v),
|
|
)
|
|
|
|
# force cast to fp32 to avoid overflowing
|
|
if _ATTN_PRECISION =="fp32":
|
|
with torch.autocast(enabled=False, device_type = 'cuda'):
|
|
q, k = q.float(), k.float()
|
|
sim = einsum('b i d, b j d -> b i j', q, k) * scale
|
|
else:
|
|
sim = einsum('b i d, b j d -> b i j', q, k) * scale
|
|
|
|
del q, k
|
|
|
|
if exists(mask):
|
|
if mask.dtype == torch.bool:
|
|
mask = rearrange(mask, 'b ... -> b (...)') #TODO: check if this bool part matches pytorch attention
|
|
max_neg_value = -torch.finfo(sim.dtype).max
|
|
mask = repeat(mask, 'b j -> (b h) () j', h=h)
|
|
sim.masked_fill_(~mask, max_neg_value)
|
|
else:
|
|
sim += mask
|
|
|
|
# attention, what we cannot get enough of
|
|
sim = sim.softmax(dim=-1)
|
|
|
|
out = einsum('b i j, b j d -> b i d', sim.to(v.dtype), v)
|
|
out = (
|
|
out.unsqueeze(0)
|
|
.reshape(b, heads, -1, dim_head)
|
|
.permute(0, 2, 1, 3)
|
|
.reshape(b, -1, heads * dim_head)
|
|
)
|
|
return out
|
|
|
|
|
|
def attention_sub_quad(query, key, value, heads, mask=None):
|
|
b, _, dim_head = query.shape
|
|
dim_head //= heads
|
|
|
|
scale = dim_head ** -0.5
|
|
query = query.unsqueeze(3).reshape(b, -1, heads, dim_head).permute(0, 2, 1, 3).reshape(b * heads, -1, dim_head)
|
|
value = value.unsqueeze(3).reshape(b, -1, heads, dim_head).permute(0, 2, 1, 3).reshape(b * heads, -1, dim_head)
|
|
|
|
key = key.unsqueeze(3).reshape(b, -1, heads, dim_head).permute(0, 2, 3, 1).reshape(b * heads, dim_head, -1)
|
|
|
|
dtype = query.dtype
|
|
upcast_attention = _ATTN_PRECISION =="fp32" and query.dtype != torch.float32
|
|
if upcast_attention:
|
|
bytes_per_token = torch.finfo(torch.float32).bits//8
|
|
else:
|
|
bytes_per_token = torch.finfo(query.dtype).bits//8
|
|
batch_x_heads, q_tokens, _ = query.shape
|
|
_, _, k_tokens = key.shape
|
|
qk_matmul_size_bytes = batch_x_heads * bytes_per_token * q_tokens * k_tokens
|
|
|
|
mem_free_total, mem_free_torch = model_management.get_free_memory(query.device, True)
|
|
|
|
kv_chunk_size_min = None
|
|
kv_chunk_size = None
|
|
query_chunk_size = None
|
|
|
|
for x in [4096, 2048, 1024, 512, 256]:
|
|
count = mem_free_total / (batch_x_heads * bytes_per_token * x * 4.0)
|
|
if count >= k_tokens:
|
|
kv_chunk_size = k_tokens
|
|
query_chunk_size = x
|
|
break
|
|
|
|
if query_chunk_size is None:
|
|
query_chunk_size = 512
|
|
|
|
hidden_states = efficient_dot_product_attention(
|
|
query,
|
|
key,
|
|
value,
|
|
query_chunk_size=query_chunk_size,
|
|
kv_chunk_size=kv_chunk_size,
|
|
kv_chunk_size_min=kv_chunk_size_min,
|
|
use_checkpoint=False,
|
|
upcast_attention=upcast_attention,
|
|
)
|
|
|
|
hidden_states = hidden_states.to(dtype)
|
|
|
|
hidden_states = hidden_states.unflatten(0, (-1, heads)).transpose(1,2).flatten(start_dim=2)
|
|
return hidden_states
|
|
|
|
def attention_split(q, k, v, heads, mask=None):
|
|
b, _, dim_head = q.shape
|
|
dim_head //= heads
|
|
scale = dim_head ** -0.5
|
|
|
|
h = heads
|
|
q, k, v = map(
|
|
lambda t: t.unsqueeze(3)
|
|
.reshape(b, -1, heads, dim_head)
|
|
.permute(0, 2, 1, 3)
|
|
.reshape(b * heads, -1, dim_head)
|
|
.contiguous(),
|
|
(q, k, v),
|
|
)
|
|
|
|
r1 = torch.zeros(q.shape[0], q.shape[1], v.shape[2], device=q.device, dtype=q.dtype)
|
|
|
|
mem_free_total = model_management.get_free_memory(q.device)
|
|
|
|
if _ATTN_PRECISION =="fp32":
|
|
element_size = 4
|
|
else:
|
|
element_size = q.element_size()
|
|
|
|
gb = 1024 ** 3
|
|
tensor_size = q.shape[0] * q.shape[1] * k.shape[1] * element_size
|
|
modifier = 3
|
|
mem_required = tensor_size * modifier
|
|
steps = 1
|
|
|
|
|
|
if mem_required > mem_free_total:
|
|
steps = 2**(math.ceil(math.log(mem_required / mem_free_total, 2)))
|
|
# print(f"Expected tensor size:{tensor_size/gb:0.1f}GB, cuda free:{mem_free_cuda/gb:0.1f}GB "
|
|
# f"torch free:{mem_free_torch/gb:0.1f} total:{mem_free_total/gb:0.1f} steps:{steps}")
|
|
|
|
if steps > 64:
|
|
max_res = math.floor(math.sqrt(math.sqrt(mem_free_total / 2.5)) / 8) * 64
|
|
raise RuntimeError(f'Not enough memory, use lower resolution (max approx. {max_res}x{max_res}). '
|
|
f'Need: {mem_required/64/gb:0.1f}GB free, Have:{mem_free_total/gb:0.1f}GB free')
|
|
|
|
# print("steps", steps, mem_required, mem_free_total, modifier, q.element_size(), tensor_size)
|
|
first_op_done = False
|
|
cleared_cache = False
|
|
while True:
|
|
try:
|
|
slice_size = q.shape[1] // steps if (q.shape[1] % steps) == 0 else q.shape[1]
|
|
for i in range(0, q.shape[1], slice_size):
|
|
end = i + slice_size
|
|
if _ATTN_PRECISION =="fp32":
|
|
with torch.autocast(enabled=False, device_type = 'cuda'):
|
|
s1 = einsum('b i d, b j d -> b i j', q[:, i:end].float(), k.float()) * scale
|
|
else:
|
|
s1 = einsum('b i d, b j d -> b i j', q[:, i:end], k) * scale
|
|
|
|
s2 = s1.softmax(dim=-1).to(v.dtype)
|
|
del s1
|
|
first_op_done = True
|
|
|
|
r1[:, i:end] = einsum('b i j, b j d -> b i d', s2, v)
|
|
del s2
|
|
break
|
|
except model_management.OOM_EXCEPTION as e:
|
|
if first_op_done == False:
|
|
model_management.soft_empty_cache(True)
|
|
if cleared_cache == False:
|
|
cleared_cache = True
|
|
print("out of memory error, emptying cache and trying again")
|
|
continue
|
|
steps *= 2
|
|
if steps > 64:
|
|
raise e
|
|
print("out of memory error, increasing steps and trying again", steps)
|
|
else:
|
|
raise e
|
|
|
|
del q, k, v
|
|
|
|
r1 = (
|
|
r1.unsqueeze(0)
|
|
.reshape(b, heads, -1, dim_head)
|
|
.permute(0, 2, 1, 3)
|
|
.reshape(b, -1, heads * dim_head)
|
|
)
|
|
return r1
|
|
|
|
BROKEN_XFORMERS = False
|
|
try:
|
|
x_vers = xformers.__version__
|
|
#I think 0.0.23 is also broken (q with bs bigger than 65535 gives CUDA error)
|
|
BROKEN_XFORMERS = x_vers.startswith("0.0.21") or x_vers.startswith("0.0.22") or x_vers.startswith("0.0.23")
|
|
except:
|
|
pass
|
|
|
|
def attention_xformers(q, k, v, heads, mask=None):
|
|
b, _, dim_head = q.shape
|
|
dim_head //= heads
|
|
if BROKEN_XFORMERS:
|
|
if b * heads > 65535:
|
|
return attention_pytorch(q, k, v, heads, mask)
|
|
|
|
q, k, v = map(
|
|
lambda t: t.unsqueeze(3)
|
|
.reshape(b, -1, heads, dim_head)
|
|
.permute(0, 2, 1, 3)
|
|
.reshape(b * heads, -1, dim_head)
|
|
.contiguous(),
|
|
(q, k, v),
|
|
)
|
|
|
|
# actually compute the attention, what we cannot get enough of
|
|
out = xformers.ops.memory_efficient_attention(q, k, v, attn_bias=None)
|
|
|
|
if exists(mask):
|
|
raise NotImplementedError
|
|
out = (
|
|
out.unsqueeze(0)
|
|
.reshape(b, heads, -1, dim_head)
|
|
.permute(0, 2, 1, 3)
|
|
.reshape(b, -1, heads * dim_head)
|
|
)
|
|
return out
|
|
|
|
def attention_pytorch(q, k, v, heads, mask=None):
|
|
b, _, dim_head = q.shape
|
|
dim_head //= heads
|
|
q, k, v = map(
|
|
lambda t: t.view(b, -1, heads, dim_head).transpose(1, 2),
|
|
(q, k, v),
|
|
)
|
|
|
|
out = torch.nn.functional.scaled_dot_product_attention(q, k, v, attn_mask=mask, dropout_p=0.0, is_causal=False)
|
|
out = (
|
|
out.transpose(1, 2).reshape(b, -1, heads * dim_head)
|
|
)
|
|
return out
|
|
|
|
|
|
optimized_attention = attention_basic
|
|
optimized_attention_masked = attention_basic
|
|
|
|
if model_management.xformers_enabled():
|
|
print("Using xformers cross attention")
|
|
optimized_attention = attention_xformers
|
|
elif model_management.pytorch_attention_enabled():
|
|
print("Using pytorch cross attention")
|
|
optimized_attention = attention_pytorch
|
|
else:
|
|
if args.use_split_cross_attention:
|
|
print("Using split optimization for cross attention")
|
|
optimized_attention = attention_split
|
|
else:
|
|
print("Using sub quadratic optimization for cross attention, if you have memory or speed issues try using: --use-split-cross-attention")
|
|
optimized_attention = attention_sub_quad
|
|
|
|
if model_management.pytorch_attention_enabled():
|
|
optimized_attention_masked = attention_pytorch
|
|
|
|
def optimized_attention_for_device(device, mask=False):
|
|
if device == torch.device("cpu"): #TODO
|
|
if model_management.pytorch_attention_enabled():
|
|
return attention_pytorch
|
|
else:
|
|
return attention_basic
|
|
if mask:
|
|
return optimized_attention_masked
|
|
|
|
return optimized_attention
|
|
|
|
|
|
class CrossAttention(nn.Module):
|
|
def __init__(self, query_dim, context_dim=None, heads=8, dim_head=64, dropout=0., dtype=None, device=None, operations=comfy.ops):
|
|
super().__init__()
|
|
inner_dim = dim_head * heads
|
|
context_dim = default(context_dim, query_dim)
|
|
|
|
self.heads = heads
|
|
self.dim_head = dim_head
|
|
|
|
self.to_q = operations.Linear(query_dim, inner_dim, bias=False, dtype=dtype, device=device)
|
|
self.to_k = operations.Linear(context_dim, inner_dim, bias=False, dtype=dtype, device=device)
|
|
self.to_v = operations.Linear(context_dim, inner_dim, bias=False, dtype=dtype, device=device)
|
|
|
|
self.to_out = nn.Sequential(operations.Linear(inner_dim, query_dim, dtype=dtype, device=device), nn.Dropout(dropout))
|
|
|
|
def forward(self, x, context=None, value=None, mask=None):
|
|
q = self.to_q(x)
|
|
context = default(context, x)
|
|
k = self.to_k(context)
|
|
if value is not None:
|
|
v = self.to_v(value)
|
|
del value
|
|
else:
|
|
v = self.to_v(context)
|
|
|
|
if mask is None:
|
|
out = optimized_attention(q, k, v, self.heads)
|
|
else:
|
|
out = optimized_attention_masked(q, k, v, self.heads, mask)
|
|
return self.to_out(out)
|
|
|
|
|
|
class BasicTransformerBlock(nn.Module):
|
|
def __init__(self, dim, n_heads, d_head, dropout=0., context_dim=None, gated_ff=True, checkpoint=True, ff_in=False, inner_dim=None,
|
|
disable_self_attn=False, disable_temporal_crossattention=False, switch_temporal_ca_to_sa=False, dtype=None, device=None, operations=comfy.ops):
|
|
super().__init__()
|
|
|
|
self.ff_in = ff_in or inner_dim is not None
|
|
if inner_dim is None:
|
|
inner_dim = dim
|
|
|
|
self.is_res = inner_dim == dim
|
|
|
|
if self.ff_in:
|
|
self.norm_in = operations.LayerNorm(dim, dtype=dtype, device=device)
|
|
self.ff_in = FeedForward(dim, dim_out=inner_dim, dropout=dropout, glu=gated_ff, dtype=dtype, device=device, operations=operations)
|
|
|
|
self.disable_self_attn = disable_self_attn
|
|
self.attn1 = CrossAttention(query_dim=inner_dim, heads=n_heads, dim_head=d_head, dropout=dropout,
|
|
context_dim=context_dim if self.disable_self_attn else None, dtype=dtype, device=device, operations=operations) # is a self-attention if not self.disable_self_attn
|
|
self.ff = FeedForward(inner_dim, dim_out=dim, dropout=dropout, glu=gated_ff, dtype=dtype, device=device, operations=operations)
|
|
|
|
if disable_temporal_crossattention:
|
|
if switch_temporal_ca_to_sa:
|
|
raise ValueError
|
|
else:
|
|
self.attn2 = None
|
|
else:
|
|
context_dim_attn2 = None
|
|
if not switch_temporal_ca_to_sa:
|
|
context_dim_attn2 = context_dim
|
|
|
|
self.attn2 = CrossAttention(query_dim=inner_dim, context_dim=context_dim_attn2,
|
|
heads=n_heads, dim_head=d_head, dropout=dropout, dtype=dtype, device=device, operations=operations) # is self-attn if context is none
|
|
self.norm2 = operations.LayerNorm(inner_dim, dtype=dtype, device=device)
|
|
|
|
self.norm1 = operations.LayerNorm(inner_dim, dtype=dtype, device=device)
|
|
self.norm3 = operations.LayerNorm(inner_dim, dtype=dtype, device=device)
|
|
self.checkpoint = checkpoint
|
|
self.n_heads = n_heads
|
|
self.d_head = d_head
|
|
self.switch_temporal_ca_to_sa = switch_temporal_ca_to_sa
|
|
|
|
def forward(self, x, context=None, transformer_options={}):
|
|
return checkpoint(self._forward, (x, context, transformer_options), self.parameters(), self.checkpoint)
|
|
|
|
def _forward(self, x, context=None, transformer_options={}):
|
|
extra_options = {}
|
|
block = transformer_options.get("block", None)
|
|
block_index = transformer_options.get("block_index", 0)
|
|
transformer_patches = {}
|
|
transformer_patches_replace = {}
|
|
|
|
for k in transformer_options:
|
|
if k == "patches":
|
|
transformer_patches = transformer_options[k]
|
|
elif k == "patches_replace":
|
|
transformer_patches_replace = transformer_options[k]
|
|
else:
|
|
extra_options[k] = transformer_options[k]
|
|
|
|
extra_options["n_heads"] = self.n_heads
|
|
extra_options["dim_head"] = self.d_head
|
|
|
|
if self.ff_in:
|
|
x_skip = x
|
|
x = self.ff_in(self.norm_in(x))
|
|
if self.is_res:
|
|
x += x_skip
|
|
|
|
n = self.norm1(x)
|
|
if self.disable_self_attn:
|
|
context_attn1 = context
|
|
else:
|
|
context_attn1 = None
|
|
value_attn1 = None
|
|
|
|
if "attn1_patch" in transformer_patches:
|
|
patch = transformer_patches["attn1_patch"]
|
|
if context_attn1 is None:
|
|
context_attn1 = n
|
|
value_attn1 = context_attn1
|
|
for p in patch:
|
|
n, context_attn1, value_attn1 = p(n, context_attn1, value_attn1, extra_options)
|
|
|
|
if block is not None:
|
|
transformer_block = (block[0], block[1], block_index)
|
|
else:
|
|
transformer_block = None
|
|
attn1_replace_patch = transformer_patches_replace.get("attn1", {})
|
|
block_attn1 = transformer_block
|
|
if block_attn1 not in attn1_replace_patch:
|
|
block_attn1 = block
|
|
|
|
if block_attn1 in attn1_replace_patch:
|
|
if context_attn1 is None:
|
|
context_attn1 = n
|
|
value_attn1 = n
|
|
n = self.attn1.to_q(n)
|
|
context_attn1 = self.attn1.to_k(context_attn1)
|
|
value_attn1 = self.attn1.to_v(value_attn1)
|
|
n = attn1_replace_patch[block_attn1](n, context_attn1, value_attn1, extra_options)
|
|
n = self.attn1.to_out(n)
|
|
else:
|
|
n = self.attn1(n, context=context_attn1, value=value_attn1)
|
|
|
|
if "attn1_output_patch" in transformer_patches:
|
|
patch = transformer_patches["attn1_output_patch"]
|
|
for p in patch:
|
|
n = p(n, extra_options)
|
|
|
|
x += n
|
|
if "middle_patch" in transformer_patches:
|
|
patch = transformer_patches["middle_patch"]
|
|
for p in patch:
|
|
x = p(x, extra_options)
|
|
|
|
if self.attn2 is not None:
|
|
n = self.norm2(x)
|
|
if self.switch_temporal_ca_to_sa:
|
|
context_attn2 = n
|
|
else:
|
|
context_attn2 = context
|
|
value_attn2 = None
|
|
if "attn2_patch" in transformer_patches:
|
|
patch = transformer_patches["attn2_patch"]
|
|
value_attn2 = context_attn2
|
|
for p in patch:
|
|
n, context_attn2, value_attn2 = p(n, context_attn2, value_attn2, extra_options)
|
|
|
|
attn2_replace_patch = transformer_patches_replace.get("attn2", {})
|
|
block_attn2 = transformer_block
|
|
if block_attn2 not in attn2_replace_patch:
|
|
block_attn2 = block
|
|
|
|
if block_attn2 in attn2_replace_patch:
|
|
if value_attn2 is None:
|
|
value_attn2 = context_attn2
|
|
n = self.attn2.to_q(n)
|
|
context_attn2 = self.attn2.to_k(context_attn2)
|
|
value_attn2 = self.attn2.to_v(value_attn2)
|
|
n = attn2_replace_patch[block_attn2](n, context_attn2, value_attn2, extra_options)
|
|
n = self.attn2.to_out(n)
|
|
else:
|
|
n = self.attn2(n, context=context_attn2, value=value_attn2)
|
|
|
|
if "attn2_output_patch" in transformer_patches:
|
|
patch = transformer_patches["attn2_output_patch"]
|
|
for p in patch:
|
|
n = p(n, extra_options)
|
|
|
|
x += n
|
|
if self.is_res:
|
|
x_skip = x
|
|
x = self.ff(self.norm3(x))
|
|
if self.is_res:
|
|
x += x_skip
|
|
|
|
return x
|
|
|
|
|
|
class SpatialTransformer(nn.Module):
|
|
"""
|
|
Transformer block for image-like data.
|
|
First, project the input (aka embedding)
|
|
and reshape to b, t, d.
|
|
Then apply standard transformer action.
|
|
Finally, reshape to image
|
|
NEW: use_linear for more efficiency instead of the 1x1 convs
|
|
"""
|
|
def __init__(self, in_channels, n_heads, d_head,
|
|
depth=1, dropout=0., context_dim=None,
|
|
disable_self_attn=False, use_linear=False,
|
|
use_checkpoint=True, dtype=None, device=None, operations=comfy.ops):
|
|
super().__init__()
|
|
if exists(context_dim) and not isinstance(context_dim, list):
|
|
context_dim = [context_dim] * depth
|
|
self.in_channels = in_channels
|
|
inner_dim = n_heads * d_head
|
|
self.norm = operations.GroupNorm(num_groups=32, num_channels=in_channels, eps=1e-6, affine=True, dtype=dtype, device=device)
|
|
if not use_linear:
|
|
self.proj_in = operations.Conv2d(in_channels,
|
|
inner_dim,
|
|
kernel_size=1,
|
|
stride=1,
|
|
padding=0, dtype=dtype, device=device)
|
|
else:
|
|
self.proj_in = operations.Linear(in_channels, inner_dim, dtype=dtype, device=device)
|
|
|
|
self.transformer_blocks = nn.ModuleList(
|
|
[BasicTransformerBlock(inner_dim, n_heads, d_head, dropout=dropout, context_dim=context_dim[d],
|
|
disable_self_attn=disable_self_attn, checkpoint=use_checkpoint, dtype=dtype, device=device, operations=operations)
|
|
for d in range(depth)]
|
|
)
|
|
if not use_linear:
|
|
self.proj_out = operations.Conv2d(inner_dim,in_channels,
|
|
kernel_size=1,
|
|
stride=1,
|
|
padding=0, dtype=dtype, device=device)
|
|
else:
|
|
self.proj_out = operations.Linear(in_channels, inner_dim, dtype=dtype, device=device)
|
|
self.use_linear = use_linear
|
|
|
|
def forward(self, x, context=None, transformer_options={}):
|
|
# note: if no context is given, cross-attention defaults to self-attention
|
|
if not isinstance(context, list):
|
|
context = [context] * len(self.transformer_blocks)
|
|
b, c, h, w = x.shape
|
|
x_in = x
|
|
x = self.norm(x)
|
|
if not self.use_linear:
|
|
x = self.proj_in(x)
|
|
x = rearrange(x, 'b c h w -> b (h w) c').contiguous()
|
|
if self.use_linear:
|
|
x = self.proj_in(x)
|
|
for i, block in enumerate(self.transformer_blocks):
|
|
transformer_options["block_index"] = i
|
|
x = block(x, context=context[i], transformer_options=transformer_options)
|
|
if self.use_linear:
|
|
x = self.proj_out(x)
|
|
x = rearrange(x, 'b (h w) c -> b c h w', h=h, w=w).contiguous()
|
|
if not self.use_linear:
|
|
x = self.proj_out(x)
|
|
return x + x_in
|
|
|
|
|
|
class SpatialVideoTransformer(SpatialTransformer):
|
|
def __init__(
|
|
self,
|
|
in_channels,
|
|
n_heads,
|
|
d_head,
|
|
depth=1,
|
|
dropout=0.0,
|
|
use_linear=False,
|
|
context_dim=None,
|
|
use_spatial_context=False,
|
|
timesteps=None,
|
|
merge_strategy: str = "fixed",
|
|
merge_factor: float = 0.5,
|
|
time_context_dim=None,
|
|
ff_in=False,
|
|
checkpoint=False,
|
|
time_depth=1,
|
|
disable_self_attn=False,
|
|
disable_temporal_crossattention=False,
|
|
max_time_embed_period: int = 10000,
|
|
dtype=None, device=None, operations=comfy.ops
|
|
):
|
|
super().__init__(
|
|
in_channels,
|
|
n_heads,
|
|
d_head,
|
|
depth=depth,
|
|
dropout=dropout,
|
|
use_checkpoint=checkpoint,
|
|
context_dim=context_dim,
|
|
use_linear=use_linear,
|
|
disable_self_attn=disable_self_attn,
|
|
dtype=dtype, device=device, operations=operations
|
|
)
|
|
self.time_depth = time_depth
|
|
self.depth = depth
|
|
self.max_time_embed_period = max_time_embed_period
|
|
|
|
time_mix_d_head = d_head
|
|
n_time_mix_heads = n_heads
|
|
|
|
time_mix_inner_dim = int(time_mix_d_head * n_time_mix_heads)
|
|
|
|
inner_dim = n_heads * d_head
|
|
if use_spatial_context:
|
|
time_context_dim = context_dim
|
|
|
|
self.time_stack = nn.ModuleList(
|
|
[
|
|
BasicTransformerBlock(
|
|
inner_dim,
|
|
n_time_mix_heads,
|
|
time_mix_d_head,
|
|
dropout=dropout,
|
|
context_dim=time_context_dim,
|
|
# timesteps=timesteps,
|
|
checkpoint=checkpoint,
|
|
ff_in=ff_in,
|
|
inner_dim=time_mix_inner_dim,
|
|
disable_self_attn=disable_self_attn,
|
|
disable_temporal_crossattention=disable_temporal_crossattention,
|
|
dtype=dtype, device=device, operations=operations
|
|
)
|
|
for _ in range(self.depth)
|
|
]
|
|
)
|
|
|
|
assert len(self.time_stack) == len(self.transformer_blocks)
|
|
|
|
self.use_spatial_context = use_spatial_context
|
|
self.in_channels = in_channels
|
|
|
|
time_embed_dim = self.in_channels * 4
|
|
self.time_pos_embed = nn.Sequential(
|
|
operations.Linear(self.in_channels, time_embed_dim, dtype=dtype, device=device),
|
|
nn.SiLU(),
|
|
operations.Linear(time_embed_dim, self.in_channels, dtype=dtype, device=device),
|
|
)
|
|
|
|
self.time_mixer = AlphaBlender(
|
|
alpha=merge_factor, merge_strategy=merge_strategy
|
|
)
|
|
|
|
def forward(
|
|
self,
|
|
x: torch.Tensor,
|
|
context: Optional[torch.Tensor] = None,
|
|
time_context: Optional[torch.Tensor] = None,
|
|
timesteps: Optional[int] = None,
|
|
image_only_indicator: Optional[torch.Tensor] = None,
|
|
transformer_options={}
|
|
) -> torch.Tensor:
|
|
_, _, h, w = x.shape
|
|
x_in = x
|
|
spatial_context = None
|
|
if exists(context):
|
|
spatial_context = context
|
|
|
|
if self.use_spatial_context:
|
|
assert (
|
|
context.ndim == 3
|
|
), f"n dims of spatial context should be 3 but are {context.ndim}"
|
|
|
|
if time_context is None:
|
|
time_context = context
|
|
time_context_first_timestep = time_context[::timesteps]
|
|
time_context = repeat(
|
|
time_context_first_timestep, "b ... -> (b n) ...", n=h * w
|
|
)
|
|
elif time_context is not None and not self.use_spatial_context:
|
|
time_context = repeat(time_context, "b ... -> (b n) ...", n=h * w)
|
|
if time_context.ndim == 2:
|
|
time_context = rearrange(time_context, "b c -> b 1 c")
|
|
|
|
x = self.norm(x)
|
|
if not self.use_linear:
|
|
x = self.proj_in(x)
|
|
x = rearrange(x, "b c h w -> b (h w) c")
|
|
if self.use_linear:
|
|
x = self.proj_in(x)
|
|
|
|
num_frames = torch.arange(timesteps, device=x.device)
|
|
num_frames = repeat(num_frames, "t -> b t", b=x.shape[0] // timesteps)
|
|
num_frames = rearrange(num_frames, "b t -> (b t)")
|
|
t_emb = timestep_embedding(num_frames, self.in_channels, repeat_only=False, max_period=self.max_time_embed_period).to(x.dtype)
|
|
emb = self.time_pos_embed(t_emb)
|
|
emb = emb[:, None, :]
|
|
|
|
for it_, (block, mix_block) in enumerate(
|
|
zip(self.transformer_blocks, self.time_stack)
|
|
):
|
|
transformer_options["block_index"] = it_
|
|
x = block(
|
|
x,
|
|
context=spatial_context,
|
|
transformer_options=transformer_options,
|
|
)
|
|
|
|
x_mix = x
|
|
x_mix = x_mix + emb
|
|
|
|
B, S, C = x_mix.shape
|
|
x_mix = rearrange(x_mix, "(b t) s c -> (b s) t c", t=timesteps)
|
|
x_mix = mix_block(x_mix, context=time_context) #TODO: transformer_options
|
|
x_mix = rearrange(
|
|
x_mix, "(b s) t c -> (b t) s c", s=S, b=B // timesteps, c=C, t=timesteps
|
|
)
|
|
|
|
x = self.time_mixer(x_spatial=x, x_temporal=x_mix, image_only_indicator=image_only_indicator)
|
|
|
|
if self.use_linear:
|
|
x = self.proj_out(x)
|
|
x = rearrange(x, "b (h w) c -> b c h w", h=h, w=w)
|
|
if not self.use_linear:
|
|
x = self.proj_out(x)
|
|
out = x + x_in
|
|
return out
|
|
|
|
|