mirror of
https://github.com/comfyanonymous/ComfyUI.git
synced 2025-01-25 15:55:18 +00:00
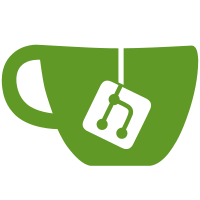
* Add clipspace feature. * feat: copy content to clipspace * feat: paste content from clipspace Extend validation to allow for validating annotated_path in addition to other parameters. Add support for annotated_filepath in folder_paths function. Generalize the '/upload/image' API to allow for uploading images to the 'input', 'temp', or 'output' directories. * rename contentClipboard -> clipspace * Do deep copy for imgs on copy to clipspace. * mask painting on clipspace * add original_imgs into clipspace * Preserve the original image when 'imgs' are modified * robust patch & refactoring folder_paths about annotated_filepath * wip * Only show the Paste menu if the ComfyApp.clipspace is not empty * clipspace feature added maskeditor feature added * instant refresh on paste force triggering 'changed' on paste action * enhance mask painting smooth drawing add brush_size +/- button * robust patch use mouseup event * robust patch again... * subfolder fix on paste logic attach subfolder if subfolder isn't empty * event listener patch add ], [ key event for brush size remove listener on close * Fix button positioning issue related to window height. Change brush size from button to slider. * clean commit * clean code * various bug fixes * paste action - prevent opening upload popup - ensure rendering after widget_value update * view api update - support annotated_filepath * maskeditor layout - prevent covering button by hidden div * remove dbg message * Add cursor functionality to display brush size * refactor: Replace brush preview feature with missionfloyd implementation * missionfloyd implementation * hiding brush preview off the canvas * change brush size on wheel event * keyup -> keydown event * Update web/extensions/core/maskeditor.js Co-authored-by: missionfloyd <missionfloyd@users.noreply.github.com> * Add support for channel-specific image data retrieval in /view API to fix alpha mask loading issue When loading an image with an alpha mask in JavaScript canvas, there is an issue where the alpha and RGB channels are premultiplied. To avoid reliance on JavaScript canvas, I added support for channel-specific image data retrieval in the "/view" API. This allows us to retrieve data for each channel separately and fix the alpha mask loading issue. The changes have been committed to the repository. * Enable brush preview for key and slider events * optimize * preview fix * robust patch * fix copy (clipspace) action imgs[0] copy -> whole imgs copy * support batch images on clipspace, maskeditor * copy/paste bug fixes for batch images enhance selector preview on clipspace menu add img_paste_mode option into clipspace menu * crash fix * print message if clipspace content cannot editable * Update web/extensions/core/maskeditor.js Co-authored-by: missionfloyd <missionfloyd@users.noreply.github.com> * make default img_paste_mode to 'selected' refactor space -> tab * save clipspace files to input/clipspace instead of temp * show "clipspace/filename.png" instead of 'filename.png [clipspace]' in LoadImage/LoadImageMask * refresh fix related to FILE_COMBO * Update web/extensions/core/maskeditor.js Co-authored-by: missionfloyd <missionfloyd@users.noreply.github.com> * Update web/extensions/core/maskeditor.js Co-authored-by: missionfloyd <missionfloyd@users.noreply.github.com> * adjust margin based on missionfloyd impelements * mouse event -> pointer event * pen, touch, mouse drawing patched and tested * Update web/extensions/core/maskeditor.js Co-authored-by: missionfloyd <missionfloyd@users.noreply.github.com> * add comment about touch event. --------- Co-authored-by: Lt.Dr.Data <lt.dr.data@gmail.com> Co-authored-by: missionfloyd <missionfloyd@users.noreply.github.com>
1303 lines
47 KiB
Python
1303 lines
47 KiB
Python
import torch
|
|
|
|
import os
|
|
import sys
|
|
import json
|
|
import hashlib
|
|
import traceback
|
|
import math
|
|
|
|
from PIL import Image
|
|
from PIL.PngImagePlugin import PngInfo
|
|
import numpy as np
|
|
|
|
|
|
sys.path.insert(0, os.path.join(os.path.dirname(os.path.realpath(__file__)), "comfy"))
|
|
|
|
|
|
import comfy.diffusers_convert
|
|
import comfy.samplers
|
|
import comfy.sample
|
|
import comfy.sd
|
|
import comfy.utils
|
|
|
|
import comfy.clip_vision
|
|
|
|
import comfy.model_management
|
|
import importlib
|
|
|
|
import folder_paths
|
|
|
|
def before_node_execution():
|
|
comfy.model_management.throw_exception_if_processing_interrupted()
|
|
|
|
def interrupt_processing(value=True):
|
|
comfy.model_management.interrupt_current_processing(value)
|
|
|
|
MAX_RESOLUTION=8192
|
|
|
|
class CLIPTextEncode:
|
|
@classmethod
|
|
def INPUT_TYPES(s):
|
|
return {"required": {"text": ("STRING", {"multiline": True}), "clip": ("CLIP", )}}
|
|
RETURN_TYPES = ("CONDITIONING",)
|
|
FUNCTION = "encode"
|
|
|
|
CATEGORY = "conditioning"
|
|
|
|
def encode(self, clip, text):
|
|
return ([[clip.encode(text), {}]], )
|
|
|
|
class ConditioningCombine:
|
|
@classmethod
|
|
def INPUT_TYPES(s):
|
|
return {"required": {"conditioning_1": ("CONDITIONING", ), "conditioning_2": ("CONDITIONING", )}}
|
|
RETURN_TYPES = ("CONDITIONING",)
|
|
FUNCTION = "combine"
|
|
|
|
CATEGORY = "conditioning"
|
|
|
|
def combine(self, conditioning_1, conditioning_2):
|
|
return (conditioning_1 + conditioning_2, )
|
|
|
|
class ConditioningAverage :
|
|
@classmethod
|
|
def INPUT_TYPES(s):
|
|
return {"required": {"conditioning_to": ("CONDITIONING", ), "conditioning_from": ("CONDITIONING", ),
|
|
"conditioning_to_strength": ("FLOAT", {"default": 1.0, "min": 0.0, "max": 1.0, "step": 0.01})
|
|
}}
|
|
RETURN_TYPES = ("CONDITIONING",)
|
|
FUNCTION = "addWeighted"
|
|
|
|
CATEGORY = "conditioning"
|
|
|
|
def addWeighted(self, conditioning_to, conditioning_from, conditioning_to_strength):
|
|
out = []
|
|
|
|
if len(conditioning_from) > 1:
|
|
print("Warning: ConditioningAverage conditioning_from contains more than 1 cond, only the first one will actually be applied to conditioning_to.")
|
|
|
|
cond_from = conditioning_from[0][0]
|
|
|
|
for i in range(len(conditioning_to)):
|
|
t1 = conditioning_to[i][0]
|
|
t0 = cond_from[:,:t1.shape[1]]
|
|
if t0.shape[1] < t1.shape[1]:
|
|
t0 = torch.cat([t0] + [torch.zeros((1, (t1.shape[1] - t0.shape[1]), t1.shape[2]))], dim=1)
|
|
|
|
tw = torch.mul(t1, conditioning_to_strength) + torch.mul(t0, (1.0 - conditioning_to_strength))
|
|
n = [tw, conditioning_to[i][1].copy()]
|
|
out.append(n)
|
|
return (out, )
|
|
|
|
class ConditioningSetArea:
|
|
@classmethod
|
|
def INPUT_TYPES(s):
|
|
return {"required": {"conditioning": ("CONDITIONING", ),
|
|
"width": ("INT", {"default": 64, "min": 64, "max": MAX_RESOLUTION, "step": 8}),
|
|
"height": ("INT", {"default": 64, "min": 64, "max": MAX_RESOLUTION, "step": 8}),
|
|
"x": ("INT", {"default": 0, "min": 0, "max": MAX_RESOLUTION, "step": 8}),
|
|
"y": ("INT", {"default": 0, "min": 0, "max": MAX_RESOLUTION, "step": 8}),
|
|
"strength": ("FLOAT", {"default": 1.0, "min": 0.0, "max": 10.0, "step": 0.01}),
|
|
}}
|
|
RETURN_TYPES = ("CONDITIONING",)
|
|
FUNCTION = "append"
|
|
|
|
CATEGORY = "conditioning"
|
|
|
|
def append(self, conditioning, width, height, x, y, strength):
|
|
c = []
|
|
for t in conditioning:
|
|
n = [t[0], t[1].copy()]
|
|
n[1]['area'] = (height // 8, width // 8, y // 8, x // 8)
|
|
n[1]['strength'] = strength
|
|
n[1]['set_area_to_bounds'] = False
|
|
c.append(n)
|
|
return (c, )
|
|
|
|
class ConditioningSetMask:
|
|
@classmethod
|
|
def INPUT_TYPES(s):
|
|
return {"required": {"conditioning": ("CONDITIONING", ),
|
|
"mask": ("MASK", ),
|
|
"strength": ("FLOAT", {"default": 1.0, "min": 0.0, "max": 10.0, "step": 0.01}),
|
|
"set_cond_area": (["default", "mask bounds"],),
|
|
}}
|
|
RETURN_TYPES = ("CONDITIONING",)
|
|
FUNCTION = "append"
|
|
|
|
CATEGORY = "conditioning"
|
|
|
|
def append(self, conditioning, mask, set_cond_area, strength):
|
|
c = []
|
|
set_area_to_bounds = False
|
|
if set_cond_area != "default":
|
|
set_area_to_bounds = True
|
|
if len(mask.shape) < 3:
|
|
mask = mask.unsqueeze(0)
|
|
for t in conditioning:
|
|
n = [t[0], t[1].copy()]
|
|
_, h, w = mask.shape
|
|
n[1]['mask'] = mask
|
|
n[1]['set_area_to_bounds'] = set_area_to_bounds
|
|
n[1]['mask_strength'] = strength
|
|
c.append(n)
|
|
return (c, )
|
|
|
|
class VAEDecode:
|
|
def __init__(self, device="cpu"):
|
|
self.device = device
|
|
|
|
@classmethod
|
|
def INPUT_TYPES(s):
|
|
return {"required": { "samples": ("LATENT", ), "vae": ("VAE", )}}
|
|
RETURN_TYPES = ("IMAGE",)
|
|
FUNCTION = "decode"
|
|
|
|
CATEGORY = "latent"
|
|
|
|
def decode(self, vae, samples):
|
|
return (vae.decode(samples["samples"]), )
|
|
|
|
class VAEDecodeTiled:
|
|
def __init__(self, device="cpu"):
|
|
self.device = device
|
|
|
|
@classmethod
|
|
def INPUT_TYPES(s):
|
|
return {"required": { "samples": ("LATENT", ), "vae": ("VAE", )}}
|
|
RETURN_TYPES = ("IMAGE",)
|
|
FUNCTION = "decode"
|
|
|
|
CATEGORY = "_for_testing"
|
|
|
|
def decode(self, vae, samples):
|
|
return (vae.decode_tiled(samples["samples"]), )
|
|
|
|
class VAEEncode:
|
|
def __init__(self, device="cpu"):
|
|
self.device = device
|
|
|
|
@classmethod
|
|
def INPUT_TYPES(s):
|
|
return {"required": { "pixels": ("IMAGE", ), "vae": ("VAE", )}}
|
|
RETURN_TYPES = ("LATENT",)
|
|
FUNCTION = "encode"
|
|
|
|
CATEGORY = "latent"
|
|
|
|
@staticmethod
|
|
def vae_encode_crop_pixels(pixels):
|
|
x = (pixels.shape[1] // 8) * 8
|
|
y = (pixels.shape[2] // 8) * 8
|
|
if pixels.shape[1] != x or pixels.shape[2] != y:
|
|
x_offset = (pixels.shape[1] % 8) // 2
|
|
y_offset = (pixels.shape[2] % 8) // 2
|
|
pixels = pixels[:, x_offset:x + x_offset, y_offset:y + y_offset, :]
|
|
return pixels
|
|
|
|
def encode(self, vae, pixels):
|
|
pixels = self.vae_encode_crop_pixels(pixels)
|
|
t = vae.encode(pixels[:,:,:,:3])
|
|
return ({"samples":t}, )
|
|
|
|
class VAEEncodeTiled:
|
|
def __init__(self, device="cpu"):
|
|
self.device = device
|
|
|
|
@classmethod
|
|
def INPUT_TYPES(s):
|
|
return {"required": { "pixels": ("IMAGE", ), "vae": ("VAE", )}}
|
|
RETURN_TYPES = ("LATENT",)
|
|
FUNCTION = "encode"
|
|
|
|
CATEGORY = "_for_testing"
|
|
|
|
def encode(self, vae, pixels):
|
|
pixels = VAEEncode.vae_encode_crop_pixels(pixels)
|
|
t = vae.encode_tiled(pixels[:,:,:,:3])
|
|
return ({"samples":t}, )
|
|
|
|
class VAEEncodeForInpaint:
|
|
def __init__(self, device="cpu"):
|
|
self.device = device
|
|
|
|
@classmethod
|
|
def INPUT_TYPES(s):
|
|
return {"required": { "pixels": ("IMAGE", ), "vae": ("VAE", ), "mask": ("MASK", ), "grow_mask_by": ("INT", {"default": 6, "min": 0, "max": 64, "step": 1}),}}
|
|
RETURN_TYPES = ("LATENT",)
|
|
FUNCTION = "encode"
|
|
|
|
CATEGORY = "latent/inpaint"
|
|
|
|
def encode(self, vae, pixels, mask, grow_mask_by=6):
|
|
x = (pixels.shape[1] // 8) * 8
|
|
y = (pixels.shape[2] // 8) * 8
|
|
mask = torch.nn.functional.interpolate(mask.reshape((-1, 1, mask.shape[-2], mask.shape[-1])), size=(pixels.shape[1], pixels.shape[2]), mode="bilinear")
|
|
|
|
pixels = pixels.clone()
|
|
if pixels.shape[1] != x or pixels.shape[2] != y:
|
|
x_offset = (pixels.shape[1] % 8) // 2
|
|
y_offset = (pixels.shape[2] % 8) // 2
|
|
pixels = pixels[:,x_offset:x + x_offset, y_offset:y + y_offset,:]
|
|
mask = mask[:,:,x_offset:x + x_offset, y_offset:y + y_offset]
|
|
|
|
#grow mask by a few pixels to keep things seamless in latent space
|
|
if grow_mask_by == 0:
|
|
mask_erosion = mask
|
|
else:
|
|
kernel_tensor = torch.ones((1, 1, grow_mask_by, grow_mask_by))
|
|
padding = math.ceil((grow_mask_by - 1) / 2)
|
|
|
|
mask_erosion = torch.clamp(torch.nn.functional.conv2d(mask.round(), kernel_tensor, padding=padding), 0, 1)
|
|
|
|
m = (1.0 - mask.round()).squeeze(1)
|
|
for i in range(3):
|
|
pixels[:,:,:,i] -= 0.5
|
|
pixels[:,:,:,i] *= m
|
|
pixels[:,:,:,i] += 0.5
|
|
t = vae.encode(pixels)
|
|
|
|
return ({"samples":t, "noise_mask": (mask_erosion[:,:,:x,:y].round())}, )
|
|
|
|
class CheckpointLoader:
|
|
@classmethod
|
|
def INPUT_TYPES(s):
|
|
return {"required": { "config_name": (folder_paths.get_filename_list("configs"), ),
|
|
"ckpt_name": (folder_paths.get_filename_list("checkpoints"), )}}
|
|
RETURN_TYPES = ("MODEL", "CLIP", "VAE")
|
|
FUNCTION = "load_checkpoint"
|
|
|
|
CATEGORY = "advanced/loaders"
|
|
|
|
def load_checkpoint(self, config_name, ckpt_name, output_vae=True, output_clip=True):
|
|
config_path = folder_paths.get_full_path("configs", config_name)
|
|
ckpt_path = folder_paths.get_full_path("checkpoints", ckpt_name)
|
|
return comfy.sd.load_checkpoint(config_path, ckpt_path, output_vae=True, output_clip=True, embedding_directory=folder_paths.get_folder_paths("embeddings"))
|
|
|
|
class CheckpointLoaderSimple:
|
|
@classmethod
|
|
def INPUT_TYPES(s):
|
|
return {"required": { "ckpt_name": (folder_paths.get_filename_list("checkpoints"), ),
|
|
}}
|
|
RETURN_TYPES = ("MODEL", "CLIP", "VAE")
|
|
FUNCTION = "load_checkpoint"
|
|
|
|
CATEGORY = "loaders"
|
|
|
|
def load_checkpoint(self, ckpt_name, output_vae=True, output_clip=True):
|
|
ckpt_path = folder_paths.get_full_path("checkpoints", ckpt_name)
|
|
out = comfy.sd.load_checkpoint_guess_config(ckpt_path, output_vae=True, output_clip=True, embedding_directory=folder_paths.get_folder_paths("embeddings"))
|
|
return out
|
|
|
|
class DiffusersLoader:
|
|
@classmethod
|
|
def INPUT_TYPES(cls):
|
|
paths = []
|
|
for search_path in folder_paths.get_folder_paths("diffusers"):
|
|
if os.path.exists(search_path):
|
|
paths += next(os.walk(search_path))[1]
|
|
return {"required": {"model_path": (paths,), }}
|
|
RETURN_TYPES = ("MODEL", "CLIP", "VAE")
|
|
FUNCTION = "load_checkpoint"
|
|
|
|
CATEGORY = "advanced/loaders"
|
|
|
|
def load_checkpoint(self, model_path, output_vae=True, output_clip=True):
|
|
for search_path in folder_paths.get_folder_paths("diffusers"):
|
|
if os.path.exists(search_path):
|
|
paths = next(os.walk(search_path))[1]
|
|
if model_path in paths:
|
|
model_path = os.path.join(search_path, model_path)
|
|
break
|
|
|
|
return comfy.diffusers_convert.load_diffusers(model_path, fp16=comfy.model_management.should_use_fp16(), output_vae=output_vae, output_clip=output_clip, embedding_directory=folder_paths.get_folder_paths("embeddings"))
|
|
|
|
|
|
class unCLIPCheckpointLoader:
|
|
@classmethod
|
|
def INPUT_TYPES(s):
|
|
return {"required": { "ckpt_name": (folder_paths.get_filename_list("checkpoints"), ),
|
|
}}
|
|
RETURN_TYPES = ("MODEL", "CLIP", "VAE", "CLIP_VISION")
|
|
FUNCTION = "load_checkpoint"
|
|
|
|
CATEGORY = "loaders"
|
|
|
|
def load_checkpoint(self, ckpt_name, output_vae=True, output_clip=True):
|
|
ckpt_path = folder_paths.get_full_path("checkpoints", ckpt_name)
|
|
out = comfy.sd.load_checkpoint_guess_config(ckpt_path, output_vae=True, output_clip=True, output_clipvision=True, embedding_directory=folder_paths.get_folder_paths("embeddings"))
|
|
return out
|
|
|
|
class CLIPSetLastLayer:
|
|
@classmethod
|
|
def INPUT_TYPES(s):
|
|
return {"required": { "clip": ("CLIP", ),
|
|
"stop_at_clip_layer": ("INT", {"default": -1, "min": -24, "max": -1, "step": 1}),
|
|
}}
|
|
RETURN_TYPES = ("CLIP",)
|
|
FUNCTION = "set_last_layer"
|
|
|
|
CATEGORY = "conditioning"
|
|
|
|
def set_last_layer(self, clip, stop_at_clip_layer):
|
|
clip = clip.clone()
|
|
clip.clip_layer(stop_at_clip_layer)
|
|
return (clip,)
|
|
|
|
class LoraLoader:
|
|
@classmethod
|
|
def INPUT_TYPES(s):
|
|
return {"required": { "model": ("MODEL",),
|
|
"clip": ("CLIP", ),
|
|
"lora_name": (folder_paths.get_filename_list("loras"), ),
|
|
"strength_model": ("FLOAT", {"default": 1.0, "min": -10.0, "max": 10.0, "step": 0.01}),
|
|
"strength_clip": ("FLOAT", {"default": 1.0, "min": -10.0, "max": 10.0, "step": 0.01}),
|
|
}}
|
|
RETURN_TYPES = ("MODEL", "CLIP")
|
|
FUNCTION = "load_lora"
|
|
|
|
CATEGORY = "loaders"
|
|
|
|
def load_lora(self, model, clip, lora_name, strength_model, strength_clip):
|
|
lora_path = folder_paths.get_full_path("loras", lora_name)
|
|
model_lora, clip_lora = comfy.sd.load_lora_for_models(model, clip, lora_path, strength_model, strength_clip)
|
|
return (model_lora, clip_lora)
|
|
|
|
class TomePatchModel:
|
|
@classmethod
|
|
def INPUT_TYPES(s):
|
|
return {"required": { "model": ("MODEL",),
|
|
"ratio": ("FLOAT", {"default": 0.3, "min": 0.0, "max": 1.0, "step": 0.01}),
|
|
}}
|
|
RETURN_TYPES = ("MODEL",)
|
|
FUNCTION = "patch"
|
|
|
|
CATEGORY = "_for_testing"
|
|
|
|
def patch(self, model, ratio):
|
|
m = model.clone()
|
|
m.set_model_tomesd(ratio)
|
|
return (m, )
|
|
|
|
class VAELoader:
|
|
@classmethod
|
|
def INPUT_TYPES(s):
|
|
return {"required": { "vae_name": (folder_paths.get_filename_list("vae"), )}}
|
|
RETURN_TYPES = ("VAE",)
|
|
FUNCTION = "load_vae"
|
|
|
|
CATEGORY = "loaders"
|
|
|
|
#TODO: scale factor?
|
|
def load_vae(self, vae_name):
|
|
vae_path = folder_paths.get_full_path("vae", vae_name)
|
|
vae = comfy.sd.VAE(ckpt_path=vae_path)
|
|
return (vae,)
|
|
|
|
class ControlNetLoader:
|
|
@classmethod
|
|
def INPUT_TYPES(s):
|
|
return {"required": { "control_net_name": (folder_paths.get_filename_list("controlnet"), )}}
|
|
|
|
RETURN_TYPES = ("CONTROL_NET",)
|
|
FUNCTION = "load_controlnet"
|
|
|
|
CATEGORY = "loaders"
|
|
|
|
def load_controlnet(self, control_net_name):
|
|
controlnet_path = folder_paths.get_full_path("controlnet", control_net_name)
|
|
controlnet = comfy.sd.load_controlnet(controlnet_path)
|
|
return (controlnet,)
|
|
|
|
class DiffControlNetLoader:
|
|
@classmethod
|
|
def INPUT_TYPES(s):
|
|
return {"required": { "model": ("MODEL",),
|
|
"control_net_name": (folder_paths.get_filename_list("controlnet"), )}}
|
|
|
|
RETURN_TYPES = ("CONTROL_NET",)
|
|
FUNCTION = "load_controlnet"
|
|
|
|
CATEGORY = "loaders"
|
|
|
|
def load_controlnet(self, model, control_net_name):
|
|
controlnet_path = folder_paths.get_full_path("controlnet", control_net_name)
|
|
controlnet = comfy.sd.load_controlnet(controlnet_path, model)
|
|
return (controlnet,)
|
|
|
|
|
|
class ControlNetApply:
|
|
@classmethod
|
|
def INPUT_TYPES(s):
|
|
return {"required": {"conditioning": ("CONDITIONING", ),
|
|
"control_net": ("CONTROL_NET", ),
|
|
"image": ("IMAGE", ),
|
|
"strength": ("FLOAT", {"default": 1.0, "min": 0.0, "max": 10.0, "step": 0.01})
|
|
}}
|
|
RETURN_TYPES = ("CONDITIONING",)
|
|
FUNCTION = "apply_controlnet"
|
|
|
|
CATEGORY = "conditioning"
|
|
|
|
def apply_controlnet(self, conditioning, control_net, image, strength):
|
|
c = []
|
|
control_hint = image.movedim(-1,1)
|
|
print(control_hint.shape)
|
|
for t in conditioning:
|
|
n = [t[0], t[1].copy()]
|
|
c_net = control_net.copy().set_cond_hint(control_hint, strength)
|
|
if 'control' in t[1]:
|
|
c_net.set_previous_controlnet(t[1]['control'])
|
|
n[1]['control'] = c_net
|
|
c.append(n)
|
|
return (c, )
|
|
|
|
class CLIPLoader:
|
|
@classmethod
|
|
def INPUT_TYPES(s):
|
|
return {"required": { "clip_name": (folder_paths.get_filename_list("clip"), ),
|
|
}}
|
|
RETURN_TYPES = ("CLIP",)
|
|
FUNCTION = "load_clip"
|
|
|
|
CATEGORY = "loaders"
|
|
|
|
def load_clip(self, clip_name):
|
|
clip_path = folder_paths.get_full_path("clip", clip_name)
|
|
clip = comfy.sd.load_clip(ckpt_path=clip_path, embedding_directory=folder_paths.get_folder_paths("embeddings"))
|
|
return (clip,)
|
|
|
|
class CLIPVisionLoader:
|
|
@classmethod
|
|
def INPUT_TYPES(s):
|
|
return {"required": { "clip_name": (folder_paths.get_filename_list("clip_vision"), ),
|
|
}}
|
|
RETURN_TYPES = ("CLIP_VISION",)
|
|
FUNCTION = "load_clip"
|
|
|
|
CATEGORY = "loaders"
|
|
|
|
def load_clip(self, clip_name):
|
|
clip_path = folder_paths.get_full_path("clip_vision", clip_name)
|
|
clip_vision = comfy.clip_vision.load(clip_path)
|
|
return (clip_vision,)
|
|
|
|
class CLIPVisionEncode:
|
|
@classmethod
|
|
def INPUT_TYPES(s):
|
|
return {"required": { "clip_vision": ("CLIP_VISION",),
|
|
"image": ("IMAGE",)
|
|
}}
|
|
RETURN_TYPES = ("CLIP_VISION_OUTPUT",)
|
|
FUNCTION = "encode"
|
|
|
|
CATEGORY = "conditioning"
|
|
|
|
def encode(self, clip_vision, image):
|
|
output = clip_vision.encode_image(image)
|
|
return (output,)
|
|
|
|
class StyleModelLoader:
|
|
@classmethod
|
|
def INPUT_TYPES(s):
|
|
return {"required": { "style_model_name": (folder_paths.get_filename_list("style_models"), )}}
|
|
|
|
RETURN_TYPES = ("STYLE_MODEL",)
|
|
FUNCTION = "load_style_model"
|
|
|
|
CATEGORY = "loaders"
|
|
|
|
def load_style_model(self, style_model_name):
|
|
style_model_path = folder_paths.get_full_path("style_models", style_model_name)
|
|
style_model = comfy.sd.load_style_model(style_model_path)
|
|
return (style_model,)
|
|
|
|
|
|
class StyleModelApply:
|
|
@classmethod
|
|
def INPUT_TYPES(s):
|
|
return {"required": {"conditioning": ("CONDITIONING", ),
|
|
"style_model": ("STYLE_MODEL", ),
|
|
"clip_vision_output": ("CLIP_VISION_OUTPUT", ),
|
|
}}
|
|
RETURN_TYPES = ("CONDITIONING",)
|
|
FUNCTION = "apply_stylemodel"
|
|
|
|
CATEGORY = "conditioning/style_model"
|
|
|
|
def apply_stylemodel(self, clip_vision_output, style_model, conditioning):
|
|
cond = style_model.get_cond(clip_vision_output)
|
|
c = []
|
|
for t in conditioning:
|
|
n = [torch.cat((t[0], cond), dim=1), t[1].copy()]
|
|
c.append(n)
|
|
return (c, )
|
|
|
|
class unCLIPConditioning:
|
|
@classmethod
|
|
def INPUT_TYPES(s):
|
|
return {"required": {"conditioning": ("CONDITIONING", ),
|
|
"clip_vision_output": ("CLIP_VISION_OUTPUT", ),
|
|
"strength": ("FLOAT", {"default": 1.0, "min": -10.0, "max": 10.0, "step": 0.01}),
|
|
"noise_augmentation": ("FLOAT", {"default": 0.0, "min": 0.0, "max": 1.0, "step": 0.01}),
|
|
}}
|
|
RETURN_TYPES = ("CONDITIONING",)
|
|
FUNCTION = "apply_adm"
|
|
|
|
CATEGORY = "conditioning"
|
|
|
|
def apply_adm(self, conditioning, clip_vision_output, strength, noise_augmentation):
|
|
c = []
|
|
for t in conditioning:
|
|
o = t[1].copy()
|
|
x = (clip_vision_output, strength, noise_augmentation)
|
|
if "adm" in o:
|
|
o["adm"] = o["adm"][:] + [x]
|
|
else:
|
|
o["adm"] = [x]
|
|
n = [t[0], o]
|
|
c.append(n)
|
|
return (c, )
|
|
|
|
class GLIGENLoader:
|
|
@classmethod
|
|
def INPUT_TYPES(s):
|
|
return {"required": { "gligen_name": (folder_paths.get_filename_list("gligen"), )}}
|
|
|
|
RETURN_TYPES = ("GLIGEN",)
|
|
FUNCTION = "load_gligen"
|
|
|
|
CATEGORY = "loaders"
|
|
|
|
def load_gligen(self, gligen_name):
|
|
gligen_path = folder_paths.get_full_path("gligen", gligen_name)
|
|
gligen = comfy.sd.load_gligen(gligen_path)
|
|
return (gligen,)
|
|
|
|
class GLIGENTextBoxApply:
|
|
@classmethod
|
|
def INPUT_TYPES(s):
|
|
return {"required": {"conditioning_to": ("CONDITIONING", ),
|
|
"clip": ("CLIP", ),
|
|
"gligen_textbox_model": ("GLIGEN", ),
|
|
"text": ("STRING", {"multiline": True}),
|
|
"width": ("INT", {"default": 64, "min": 8, "max": MAX_RESOLUTION, "step": 8}),
|
|
"height": ("INT", {"default": 64, "min": 8, "max": MAX_RESOLUTION, "step": 8}),
|
|
"x": ("INT", {"default": 0, "min": 0, "max": MAX_RESOLUTION, "step": 8}),
|
|
"y": ("INT", {"default": 0, "min": 0, "max": MAX_RESOLUTION, "step": 8}),
|
|
}}
|
|
RETURN_TYPES = ("CONDITIONING",)
|
|
FUNCTION = "append"
|
|
|
|
CATEGORY = "conditioning/gligen"
|
|
|
|
def append(self, conditioning_to, clip, gligen_textbox_model, text, width, height, x, y):
|
|
c = []
|
|
cond, cond_pooled = clip.encode_from_tokens(clip.tokenize(text), return_pooled=True)
|
|
for t in conditioning_to:
|
|
n = [t[0], t[1].copy()]
|
|
position_params = [(cond_pooled, height // 8, width // 8, y // 8, x // 8)]
|
|
prev = []
|
|
if "gligen" in n[1]:
|
|
prev = n[1]['gligen'][2]
|
|
|
|
n[1]['gligen'] = ("position", gligen_textbox_model, prev + position_params)
|
|
c.append(n)
|
|
return (c, )
|
|
|
|
class EmptyLatentImage:
|
|
def __init__(self, device="cpu"):
|
|
self.device = device
|
|
|
|
@classmethod
|
|
def INPUT_TYPES(s):
|
|
return {"required": { "width": ("INT", {"default": 512, "min": 64, "max": MAX_RESOLUTION, "step": 8}),
|
|
"height": ("INT", {"default": 512, "min": 64, "max": MAX_RESOLUTION, "step": 8}),
|
|
"batch_size": ("INT", {"default": 1, "min": 1, "max": 64})}}
|
|
RETURN_TYPES = ("LATENT",)
|
|
FUNCTION = "generate"
|
|
|
|
CATEGORY = "latent"
|
|
|
|
def generate(self, width, height, batch_size=1):
|
|
latent = torch.zeros([batch_size, 4, height // 8, width // 8])
|
|
return ({"samples":latent}, )
|
|
|
|
|
|
class LatentFromBatch:
|
|
@classmethod
|
|
def INPUT_TYPES(s):
|
|
return {"required": { "samples": ("LATENT",),
|
|
"batch_index": ("INT", {"default": 0, "min": 0, "max": 63}),
|
|
}}
|
|
RETURN_TYPES = ("LATENT",)
|
|
FUNCTION = "rotate"
|
|
|
|
CATEGORY = "latent"
|
|
|
|
def rotate(self, samples, batch_index):
|
|
s = samples.copy()
|
|
s_in = samples["samples"]
|
|
batch_index = min(s_in.shape[0] - 1, batch_index)
|
|
s["samples"] = s_in[batch_index:batch_index + 1].clone()
|
|
s["batch_index"] = batch_index
|
|
return (s,)
|
|
|
|
class LatentUpscale:
|
|
upscale_methods = ["nearest-exact", "bilinear", "area"]
|
|
crop_methods = ["disabled", "center"]
|
|
|
|
@classmethod
|
|
def INPUT_TYPES(s):
|
|
return {"required": { "samples": ("LATENT",), "upscale_method": (s.upscale_methods,),
|
|
"width": ("INT", {"default": 512, "min": 64, "max": MAX_RESOLUTION, "step": 8}),
|
|
"height": ("INT", {"default": 512, "min": 64, "max": MAX_RESOLUTION, "step": 8}),
|
|
"crop": (s.crop_methods,)}}
|
|
RETURN_TYPES = ("LATENT",)
|
|
FUNCTION = "upscale"
|
|
|
|
CATEGORY = "latent"
|
|
|
|
def upscale(self, samples, upscale_method, width, height, crop):
|
|
s = samples.copy()
|
|
s["samples"] = comfy.utils.common_upscale(samples["samples"], width // 8, height // 8, upscale_method, crop)
|
|
return (s,)
|
|
|
|
class LatentRotate:
|
|
@classmethod
|
|
def INPUT_TYPES(s):
|
|
return {"required": { "samples": ("LATENT",),
|
|
"rotation": (["none", "90 degrees", "180 degrees", "270 degrees"],),
|
|
}}
|
|
RETURN_TYPES = ("LATENT",)
|
|
FUNCTION = "rotate"
|
|
|
|
CATEGORY = "latent/transform"
|
|
|
|
def rotate(self, samples, rotation):
|
|
s = samples.copy()
|
|
rotate_by = 0
|
|
if rotation.startswith("90"):
|
|
rotate_by = 1
|
|
elif rotation.startswith("180"):
|
|
rotate_by = 2
|
|
elif rotation.startswith("270"):
|
|
rotate_by = 3
|
|
|
|
s["samples"] = torch.rot90(samples["samples"], k=rotate_by, dims=[3, 2])
|
|
return (s,)
|
|
|
|
class LatentFlip:
|
|
@classmethod
|
|
def INPUT_TYPES(s):
|
|
return {"required": { "samples": ("LATENT",),
|
|
"flip_method": (["x-axis: vertically", "y-axis: horizontally"],),
|
|
}}
|
|
RETURN_TYPES = ("LATENT",)
|
|
FUNCTION = "flip"
|
|
|
|
CATEGORY = "latent/transform"
|
|
|
|
def flip(self, samples, flip_method):
|
|
s = samples.copy()
|
|
if flip_method.startswith("x"):
|
|
s["samples"] = torch.flip(samples["samples"], dims=[2])
|
|
elif flip_method.startswith("y"):
|
|
s["samples"] = torch.flip(samples["samples"], dims=[3])
|
|
|
|
return (s,)
|
|
|
|
class LatentComposite:
|
|
@classmethod
|
|
def INPUT_TYPES(s):
|
|
return {"required": { "samples_to": ("LATENT",),
|
|
"samples_from": ("LATENT",),
|
|
"x": ("INT", {"default": 0, "min": 0, "max": MAX_RESOLUTION, "step": 8}),
|
|
"y": ("INT", {"default": 0, "min": 0, "max": MAX_RESOLUTION, "step": 8}),
|
|
"feather": ("INT", {"default": 0, "min": 0, "max": MAX_RESOLUTION, "step": 8}),
|
|
}}
|
|
RETURN_TYPES = ("LATENT",)
|
|
FUNCTION = "composite"
|
|
|
|
CATEGORY = "latent"
|
|
|
|
def composite(self, samples_to, samples_from, x, y, composite_method="normal", feather=0):
|
|
x = x // 8
|
|
y = y // 8
|
|
feather = feather // 8
|
|
samples_out = samples_to.copy()
|
|
s = samples_to["samples"].clone()
|
|
samples_to = samples_to["samples"]
|
|
samples_from = samples_from["samples"]
|
|
if feather == 0:
|
|
s[:,:,y:y+samples_from.shape[2],x:x+samples_from.shape[3]] = samples_from[:,:,:samples_to.shape[2] - y, :samples_to.shape[3] - x]
|
|
else:
|
|
samples_from = samples_from[:,:,:samples_to.shape[2] - y, :samples_to.shape[3] - x]
|
|
mask = torch.ones_like(samples_from)
|
|
for t in range(feather):
|
|
if y != 0:
|
|
mask[:,:,t:1+t,:] *= ((1.0/feather) * (t + 1))
|
|
|
|
if y + samples_from.shape[2] < samples_to.shape[2]:
|
|
mask[:,:,mask.shape[2] -1 -t: mask.shape[2]-t,:] *= ((1.0/feather) * (t + 1))
|
|
if x != 0:
|
|
mask[:,:,:,t:1+t] *= ((1.0/feather) * (t + 1))
|
|
if x + samples_from.shape[3] < samples_to.shape[3]:
|
|
mask[:,:,:,mask.shape[3]- 1 - t: mask.shape[3]- t] *= ((1.0/feather) * (t + 1))
|
|
rev_mask = torch.ones_like(mask) - mask
|
|
s[:,:,y:y+samples_from.shape[2],x:x+samples_from.shape[3]] = samples_from[:,:,:samples_to.shape[2] - y, :samples_to.shape[3] - x] * mask + s[:,:,y:y+samples_from.shape[2],x:x+samples_from.shape[3]] * rev_mask
|
|
samples_out["samples"] = s
|
|
return (samples_out,)
|
|
|
|
class LatentCrop:
|
|
@classmethod
|
|
def INPUT_TYPES(s):
|
|
return {"required": { "samples": ("LATENT",),
|
|
"width": ("INT", {"default": 512, "min": 64, "max": MAX_RESOLUTION, "step": 8}),
|
|
"height": ("INT", {"default": 512, "min": 64, "max": MAX_RESOLUTION, "step": 8}),
|
|
"x": ("INT", {"default": 0, "min": 0, "max": MAX_RESOLUTION, "step": 8}),
|
|
"y": ("INT", {"default": 0, "min": 0, "max": MAX_RESOLUTION, "step": 8}),
|
|
}}
|
|
RETURN_TYPES = ("LATENT",)
|
|
FUNCTION = "crop"
|
|
|
|
CATEGORY = "latent/transform"
|
|
|
|
def crop(self, samples, width, height, x, y):
|
|
s = samples.copy()
|
|
samples = samples['samples']
|
|
x = x // 8
|
|
y = y // 8
|
|
|
|
#enfonce minimum size of 64
|
|
if x > (samples.shape[3] - 8):
|
|
x = samples.shape[3] - 8
|
|
if y > (samples.shape[2] - 8):
|
|
y = samples.shape[2] - 8
|
|
|
|
new_height = height // 8
|
|
new_width = width // 8
|
|
to_x = new_width + x
|
|
to_y = new_height + y
|
|
s['samples'] = samples[:,:,y:to_y, x:to_x]
|
|
return (s,)
|
|
|
|
class SetLatentNoiseMask:
|
|
@classmethod
|
|
def INPUT_TYPES(s):
|
|
return {"required": { "samples": ("LATENT",),
|
|
"mask": ("MASK",),
|
|
}}
|
|
RETURN_TYPES = ("LATENT",)
|
|
FUNCTION = "set_mask"
|
|
|
|
CATEGORY = "latent/inpaint"
|
|
|
|
def set_mask(self, samples, mask):
|
|
s = samples.copy()
|
|
s["noise_mask"] = mask
|
|
return (s,)
|
|
|
|
def common_ksampler(model, seed, steps, cfg, sampler_name, scheduler, positive, negative, latent, denoise=1.0, disable_noise=False, start_step=None, last_step=None, force_full_denoise=False):
|
|
device = comfy.model_management.get_torch_device()
|
|
latent_image = latent["samples"]
|
|
|
|
if disable_noise:
|
|
noise = torch.zeros(latent_image.size(), dtype=latent_image.dtype, layout=latent_image.layout, device="cpu")
|
|
else:
|
|
skip = latent["batch_index"] if "batch_index" in latent else 0
|
|
noise = comfy.sample.prepare_noise(latent_image, seed, skip)
|
|
|
|
noise_mask = None
|
|
if "noise_mask" in latent:
|
|
noise_mask = latent["noise_mask"]
|
|
|
|
pbar = comfy.utils.ProgressBar(steps)
|
|
def callback(step, x0, x, total_steps):
|
|
pbar.update_absolute(step + 1, total_steps)
|
|
|
|
samples = comfy.sample.sample(model, noise, steps, cfg, sampler_name, scheduler, positive, negative, latent_image,
|
|
denoise=denoise, disable_noise=disable_noise, start_step=start_step, last_step=last_step,
|
|
force_full_denoise=force_full_denoise, noise_mask=noise_mask, callback=callback)
|
|
out = latent.copy()
|
|
out["samples"] = samples
|
|
return (out, )
|
|
|
|
class KSampler:
|
|
@classmethod
|
|
def INPUT_TYPES(s):
|
|
return {"required":
|
|
{"model": ("MODEL",),
|
|
"seed": ("INT", {"default": 0, "min": 0, "max": 0xffffffffffffffff}),
|
|
"steps": ("INT", {"default": 20, "min": 1, "max": 10000}),
|
|
"cfg": ("FLOAT", {"default": 8.0, "min": 0.0, "max": 100.0}),
|
|
"sampler_name": (comfy.samplers.KSampler.SAMPLERS, ),
|
|
"scheduler": (comfy.samplers.KSampler.SCHEDULERS, ),
|
|
"positive": ("CONDITIONING", ),
|
|
"negative": ("CONDITIONING", ),
|
|
"latent_image": ("LATENT", ),
|
|
"denoise": ("FLOAT", {"default": 1.0, "min": 0.0, "max": 1.0, "step": 0.01}),
|
|
}}
|
|
|
|
RETURN_TYPES = ("LATENT",)
|
|
FUNCTION = "sample"
|
|
|
|
CATEGORY = "sampling"
|
|
|
|
def sample(self, model, seed, steps, cfg, sampler_name, scheduler, positive, negative, latent_image, denoise=1.0):
|
|
return common_ksampler(model, seed, steps, cfg, sampler_name, scheduler, positive, negative, latent_image, denoise=denoise)
|
|
|
|
class KSamplerAdvanced:
|
|
@classmethod
|
|
def INPUT_TYPES(s):
|
|
return {"required":
|
|
{"model": ("MODEL",),
|
|
"add_noise": (["enable", "disable"], ),
|
|
"noise_seed": ("INT", {"default": 0, "min": 0, "max": 0xffffffffffffffff}),
|
|
"steps": ("INT", {"default": 20, "min": 1, "max": 10000}),
|
|
"cfg": ("FLOAT", {"default": 8.0, "min": 0.0, "max": 100.0}),
|
|
"sampler_name": (comfy.samplers.KSampler.SAMPLERS, ),
|
|
"scheduler": (comfy.samplers.KSampler.SCHEDULERS, ),
|
|
"positive": ("CONDITIONING", ),
|
|
"negative": ("CONDITIONING", ),
|
|
"latent_image": ("LATENT", ),
|
|
"start_at_step": ("INT", {"default": 0, "min": 0, "max": 10000}),
|
|
"end_at_step": ("INT", {"default": 10000, "min": 0, "max": 10000}),
|
|
"return_with_leftover_noise": (["disable", "enable"], ),
|
|
}}
|
|
|
|
RETURN_TYPES = ("LATENT",)
|
|
FUNCTION = "sample"
|
|
|
|
CATEGORY = "sampling"
|
|
|
|
def sample(self, model, add_noise, noise_seed, steps, cfg, sampler_name, scheduler, positive, negative, latent_image, start_at_step, end_at_step, return_with_leftover_noise, denoise=1.0):
|
|
force_full_denoise = True
|
|
if return_with_leftover_noise == "enable":
|
|
force_full_denoise = False
|
|
disable_noise = False
|
|
if add_noise == "disable":
|
|
disable_noise = True
|
|
return common_ksampler(model, noise_seed, steps, cfg, sampler_name, scheduler, positive, negative, latent_image, denoise=denoise, disable_noise=disable_noise, start_step=start_at_step, last_step=end_at_step, force_full_denoise=force_full_denoise)
|
|
|
|
class SaveImage:
|
|
def __init__(self):
|
|
self.output_dir = folder_paths.get_output_directory()
|
|
self.type = "output"
|
|
|
|
@classmethod
|
|
def INPUT_TYPES(s):
|
|
return {"required":
|
|
{"images": ("IMAGE", ),
|
|
"filename_prefix": ("STRING", {"default": "ComfyUI"})},
|
|
"hidden": {"prompt": "PROMPT", "extra_pnginfo": "EXTRA_PNGINFO"},
|
|
}
|
|
|
|
RETURN_TYPES = ()
|
|
FUNCTION = "save_images"
|
|
|
|
OUTPUT_NODE = True
|
|
|
|
CATEGORY = "image"
|
|
|
|
def save_images(self, images, filename_prefix="ComfyUI", prompt=None, extra_pnginfo=None):
|
|
def map_filename(filename):
|
|
prefix_len = len(os.path.basename(filename_prefix))
|
|
prefix = filename[:prefix_len + 1]
|
|
try:
|
|
digits = int(filename[prefix_len + 1:].split('_')[0])
|
|
except:
|
|
digits = 0
|
|
return (digits, prefix)
|
|
|
|
def compute_vars(input):
|
|
input = input.replace("%width%", str(images[0].shape[1]))
|
|
input = input.replace("%height%", str(images[0].shape[0]))
|
|
return input
|
|
|
|
filename_prefix = compute_vars(filename_prefix)
|
|
|
|
subfolder = os.path.dirname(os.path.normpath(filename_prefix))
|
|
filename = os.path.basename(os.path.normpath(filename_prefix))
|
|
|
|
full_output_folder = os.path.join(self.output_dir, subfolder)
|
|
|
|
if os.path.commonpath((self.output_dir, os.path.abspath(full_output_folder))) != self.output_dir:
|
|
print("Saving image outside the output folder is not allowed.")
|
|
return {}
|
|
|
|
try:
|
|
counter = max(filter(lambda a: a[1][:-1] == filename and a[1][-1] == "_", map(map_filename, os.listdir(full_output_folder))))[0] + 1
|
|
except ValueError:
|
|
counter = 1
|
|
except FileNotFoundError:
|
|
os.makedirs(full_output_folder, exist_ok=True)
|
|
counter = 1
|
|
|
|
results = list()
|
|
for image in images:
|
|
i = 255. * image.cpu().numpy()
|
|
img = Image.fromarray(np.clip(i, 0, 255).astype(np.uint8))
|
|
metadata = PngInfo()
|
|
if prompt is not None:
|
|
metadata.add_text("prompt", json.dumps(prompt))
|
|
if extra_pnginfo is not None:
|
|
for x in extra_pnginfo:
|
|
metadata.add_text(x, json.dumps(extra_pnginfo[x]))
|
|
|
|
file = f"{filename}_{counter:05}_.png"
|
|
img.save(os.path.join(full_output_folder, file), pnginfo=metadata, compress_level=4)
|
|
results.append({
|
|
"filename": file,
|
|
"subfolder": subfolder,
|
|
"type": self.type
|
|
})
|
|
counter += 1
|
|
|
|
return { "ui": { "images": results } }
|
|
|
|
class PreviewImage(SaveImage):
|
|
def __init__(self):
|
|
self.output_dir = folder_paths.get_temp_directory()
|
|
self.type = "temp"
|
|
|
|
@classmethod
|
|
def INPUT_TYPES(s):
|
|
return {"required":
|
|
{"images": ("IMAGE", ), },
|
|
"hidden": {"prompt": "PROMPT", "extra_pnginfo": "EXTRA_PNGINFO"},
|
|
}
|
|
|
|
class LoadImage:
|
|
@classmethod
|
|
def INPUT_TYPES(s):
|
|
input_dir = folder_paths.get_input_directory()
|
|
input_dir = [f for f in os.listdir(input_dir) if os.path.isfile(os.path.join(input_dir, f))]
|
|
return {"required":
|
|
{"image": ("FILE_COMBO", {"base_dir": "input", "files": sorted(input_dir)}, )},
|
|
}
|
|
|
|
CATEGORY = "image"
|
|
|
|
RETURN_TYPES = ("IMAGE", "MASK")
|
|
FUNCTION = "load_image"
|
|
def load_image(self, image):
|
|
image_path = folder_paths.get_annotated_filepath(image)
|
|
i = Image.open(image_path)
|
|
image = i.convert("RGB")
|
|
image = np.array(image).astype(np.float32) / 255.0
|
|
image = torch.from_numpy(image)[None,]
|
|
if 'A' in i.getbands():
|
|
mask = np.array(i.getchannel('A')).astype(np.float32) / 255.0
|
|
mask = 1. - torch.from_numpy(mask)
|
|
else:
|
|
mask = torch.zeros((64,64), dtype=torch.float32, device="cpu")
|
|
return (image, mask)
|
|
|
|
@classmethod
|
|
def IS_CHANGED(s, image):
|
|
image_path = folder_paths.get_annotated_filepath(image)
|
|
m = hashlib.sha256()
|
|
with open(image_path, 'rb') as f:
|
|
m.update(f.read())
|
|
return m.digest().hex()
|
|
|
|
@classmethod
|
|
def VALIDATE_INPUTS(s, image):
|
|
if not folder_paths.exists_annotated_filepath(image):
|
|
return "Invalid image file: {}".format(image)
|
|
|
|
return True
|
|
|
|
class LoadImageMask:
|
|
_color_channels = ["alpha", "red", "green", "blue"]
|
|
@classmethod
|
|
def INPUT_TYPES(s):
|
|
input_dir = folder_paths.get_input_directory()
|
|
input_dir = [f for f in os.listdir(input_dir) if os.path.isfile(os.path.join(input_dir, f))]
|
|
return {"required":
|
|
{"image": ("FILE_COMBO", {"base_dir": "input", "files": sorted(input_dir)}, ),
|
|
"channel": (s._color_channels, ), }
|
|
}
|
|
|
|
CATEGORY = "mask"
|
|
|
|
RETURN_TYPES = ("MASK",)
|
|
FUNCTION = "load_image"
|
|
def load_image(self, image, channel):
|
|
image_path = folder_paths.get_annotated_filepath(image)
|
|
i = Image.open(image_path)
|
|
if i.getbands() != ("R", "G", "B", "A"):
|
|
i = i.convert("RGBA")
|
|
mask = None
|
|
c = channel[0].upper()
|
|
if c in i.getbands():
|
|
mask = np.array(i.getchannel(c)).astype(np.float32) / 255.0
|
|
mask = torch.from_numpy(mask)
|
|
if c == 'A':
|
|
mask = 1. - mask
|
|
else:
|
|
mask = torch.zeros((64,64), dtype=torch.float32, device="cpu")
|
|
return (mask,)
|
|
|
|
@classmethod
|
|
def IS_CHANGED(s, image, channel):
|
|
image_path = folder_paths.get_annotated_filepath(image)
|
|
m = hashlib.sha256()
|
|
with open(image_path, 'rb') as f:
|
|
m.update(f.read())
|
|
return m.digest().hex()
|
|
|
|
@classmethod
|
|
def VALIDATE_INPUTS(s, image, channel):
|
|
if not folder_paths.exists_annotated_filepath(image):
|
|
return "Invalid image file: {}".format(image)
|
|
|
|
if channel not in s._color_channels:
|
|
return "Invalid color channel: {}".format(channel)
|
|
|
|
return True
|
|
|
|
class ImageScale:
|
|
upscale_methods = ["nearest-exact", "bilinear", "area"]
|
|
crop_methods = ["disabled", "center"]
|
|
|
|
@classmethod
|
|
def INPUT_TYPES(s):
|
|
return {"required": { "image": ("IMAGE",), "upscale_method": (s.upscale_methods,),
|
|
"width": ("INT", {"default": 512, "min": 1, "max": MAX_RESOLUTION, "step": 1}),
|
|
"height": ("INT", {"default": 512, "min": 1, "max": MAX_RESOLUTION, "step": 1}),
|
|
"crop": (s.crop_methods,)}}
|
|
RETURN_TYPES = ("IMAGE",)
|
|
FUNCTION = "upscale"
|
|
|
|
CATEGORY = "image/upscaling"
|
|
|
|
def upscale(self, image, upscale_method, width, height, crop):
|
|
samples = image.movedim(-1,1)
|
|
s = comfy.utils.common_upscale(samples, width, height, upscale_method, crop)
|
|
s = s.movedim(1,-1)
|
|
return (s,)
|
|
|
|
class ImageInvert:
|
|
|
|
@classmethod
|
|
def INPUT_TYPES(s):
|
|
return {"required": { "image": ("IMAGE",)}}
|
|
|
|
RETURN_TYPES = ("IMAGE",)
|
|
FUNCTION = "invert"
|
|
|
|
CATEGORY = "image"
|
|
|
|
def invert(self, image):
|
|
s = 1.0 - image
|
|
return (s,)
|
|
|
|
|
|
class ImagePadForOutpaint:
|
|
|
|
@classmethod
|
|
def INPUT_TYPES(s):
|
|
return {
|
|
"required": {
|
|
"image": ("IMAGE",),
|
|
"left": ("INT", {"default": 0, "min": 0, "max": MAX_RESOLUTION, "step": 8}),
|
|
"top": ("INT", {"default": 0, "min": 0, "max": MAX_RESOLUTION, "step": 8}),
|
|
"right": ("INT", {"default": 0, "min": 0, "max": MAX_RESOLUTION, "step": 8}),
|
|
"bottom": ("INT", {"default": 0, "min": 0, "max": MAX_RESOLUTION, "step": 8}),
|
|
"feathering": ("INT", {"default": 40, "min": 0, "max": MAX_RESOLUTION, "step": 1}),
|
|
}
|
|
}
|
|
|
|
RETURN_TYPES = ("IMAGE", "MASK")
|
|
FUNCTION = "expand_image"
|
|
|
|
CATEGORY = "image"
|
|
|
|
def expand_image(self, image, left, top, right, bottom, feathering):
|
|
d1, d2, d3, d4 = image.size()
|
|
|
|
new_image = torch.zeros(
|
|
(d1, d2 + top + bottom, d3 + left + right, d4),
|
|
dtype=torch.float32,
|
|
)
|
|
new_image[:, top:top + d2, left:left + d3, :] = image
|
|
|
|
mask = torch.ones(
|
|
(d2 + top + bottom, d3 + left + right),
|
|
dtype=torch.float32,
|
|
)
|
|
|
|
t = torch.zeros(
|
|
(d2, d3),
|
|
dtype=torch.float32
|
|
)
|
|
|
|
if feathering > 0 and feathering * 2 < d2 and feathering * 2 < d3:
|
|
|
|
for i in range(d2):
|
|
for j in range(d3):
|
|
dt = i if top != 0 else d2
|
|
db = d2 - i if bottom != 0 else d2
|
|
|
|
dl = j if left != 0 else d3
|
|
dr = d3 - j if right != 0 else d3
|
|
|
|
d = min(dt, db, dl, dr)
|
|
|
|
if d >= feathering:
|
|
continue
|
|
|
|
v = (feathering - d) / feathering
|
|
|
|
t[i, j] = v * v
|
|
|
|
mask[top:top + d2, left:left + d3] = t
|
|
|
|
return (new_image, mask)
|
|
|
|
|
|
NODE_CLASS_MAPPINGS = {
|
|
"KSampler": KSampler,
|
|
"CheckpointLoaderSimple": CheckpointLoaderSimple,
|
|
"CLIPTextEncode": CLIPTextEncode,
|
|
"CLIPSetLastLayer": CLIPSetLastLayer,
|
|
"VAEDecode": VAEDecode,
|
|
"VAEEncode": VAEEncode,
|
|
"VAEEncodeForInpaint": VAEEncodeForInpaint,
|
|
"VAELoader": VAELoader,
|
|
"EmptyLatentImage": EmptyLatentImage,
|
|
"LatentUpscale": LatentUpscale,
|
|
"LatentFromBatch": LatentFromBatch,
|
|
"SaveImage": SaveImage,
|
|
"PreviewImage": PreviewImage,
|
|
"LoadImage": LoadImage,
|
|
"LoadImageMask": LoadImageMask,
|
|
"ImageScale": ImageScale,
|
|
"ImageInvert": ImageInvert,
|
|
"ImagePadForOutpaint": ImagePadForOutpaint,
|
|
"ConditioningAverage ": ConditioningAverage ,
|
|
"ConditioningCombine": ConditioningCombine,
|
|
"ConditioningSetArea": ConditioningSetArea,
|
|
"ConditioningSetMask": ConditioningSetMask,
|
|
"KSamplerAdvanced": KSamplerAdvanced,
|
|
"SetLatentNoiseMask": SetLatentNoiseMask,
|
|
"LatentComposite": LatentComposite,
|
|
"LatentRotate": LatentRotate,
|
|
"LatentFlip": LatentFlip,
|
|
"LatentCrop": LatentCrop,
|
|
"LoraLoader": LoraLoader,
|
|
"CLIPLoader": CLIPLoader,
|
|
"CLIPVisionEncode": CLIPVisionEncode,
|
|
"StyleModelApply": StyleModelApply,
|
|
"unCLIPConditioning": unCLIPConditioning,
|
|
"ControlNetApply": ControlNetApply,
|
|
"ControlNetLoader": ControlNetLoader,
|
|
"DiffControlNetLoader": DiffControlNetLoader,
|
|
"StyleModelLoader": StyleModelLoader,
|
|
"CLIPVisionLoader": CLIPVisionLoader,
|
|
"VAEDecodeTiled": VAEDecodeTiled,
|
|
"VAEEncodeTiled": VAEEncodeTiled,
|
|
"TomePatchModel": TomePatchModel,
|
|
"unCLIPCheckpointLoader": unCLIPCheckpointLoader,
|
|
"GLIGENLoader": GLIGENLoader,
|
|
"GLIGENTextBoxApply": GLIGENTextBoxApply,
|
|
|
|
"CheckpointLoader": CheckpointLoader,
|
|
"DiffusersLoader": DiffusersLoader,
|
|
}
|
|
|
|
NODE_DISPLAY_NAME_MAPPINGS = {
|
|
# Sampling
|
|
"KSampler": "KSampler",
|
|
"KSamplerAdvanced": "KSampler (Advanced)",
|
|
# Loaders
|
|
"CheckpointLoader": "Load Checkpoint (With Config)",
|
|
"CheckpointLoaderSimple": "Load Checkpoint",
|
|
"VAELoader": "Load VAE",
|
|
"LoraLoader": "Load LoRA",
|
|
"CLIPLoader": "Load CLIP",
|
|
"ControlNetLoader": "Load ControlNet Model",
|
|
"DiffControlNetLoader": "Load ControlNet Model (diff)",
|
|
"StyleModelLoader": "Load Style Model",
|
|
"CLIPVisionLoader": "Load CLIP Vision",
|
|
"UpscaleModelLoader": "Load Upscale Model",
|
|
# Conditioning
|
|
"CLIPVisionEncode": "CLIP Vision Encode",
|
|
"StyleModelApply": "Apply Style Model",
|
|
"CLIPTextEncode": "CLIP Text Encode (Prompt)",
|
|
"CLIPSetLastLayer": "CLIP Set Last Layer",
|
|
"ConditioningCombine": "Conditioning (Combine)",
|
|
"ConditioningAverage ": "Conditioning (Average)",
|
|
"ConditioningSetArea": "Conditioning (Set Area)",
|
|
"ConditioningSetMask": "Conditioning (Set Mask)",
|
|
"ControlNetApply": "Apply ControlNet",
|
|
# Latent
|
|
"VAEEncodeForInpaint": "VAE Encode (for Inpainting)",
|
|
"SetLatentNoiseMask": "Set Latent Noise Mask",
|
|
"VAEDecode": "VAE Decode",
|
|
"VAEEncode": "VAE Encode",
|
|
"LatentRotate": "Rotate Latent",
|
|
"LatentFlip": "Flip Latent",
|
|
"LatentCrop": "Crop Latent",
|
|
"EmptyLatentImage": "Empty Latent Image",
|
|
"LatentUpscale": "Upscale Latent",
|
|
"LatentComposite": "Latent Composite",
|
|
# Image
|
|
"SaveImage": "Save Image",
|
|
"PreviewImage": "Preview Image",
|
|
"LoadImage": "Load Image",
|
|
"LoadImageMask": "Load Image (as Mask)",
|
|
"ImageScale": "Upscale Image",
|
|
"ImageUpscaleWithModel": "Upscale Image (using Model)",
|
|
"ImageInvert": "Invert Image",
|
|
"ImagePadForOutpaint": "Pad Image for Outpainting",
|
|
# _for_testing
|
|
"VAEDecodeTiled": "VAE Decode (Tiled)",
|
|
"VAEEncodeTiled": "VAE Encode (Tiled)",
|
|
}
|
|
|
|
def load_custom_node(module_path):
|
|
module_name = os.path.basename(module_path)
|
|
if os.path.isfile(module_path):
|
|
sp = os.path.splitext(module_path)
|
|
module_name = sp[0]
|
|
try:
|
|
if os.path.isfile(module_path):
|
|
module_spec = importlib.util.spec_from_file_location(module_name, module_path)
|
|
else:
|
|
module_spec = importlib.util.spec_from_file_location(module_name, os.path.join(module_path, "__init__.py"))
|
|
module = importlib.util.module_from_spec(module_spec)
|
|
sys.modules[module_name] = module
|
|
module_spec.loader.exec_module(module)
|
|
if hasattr(module, "NODE_CLASS_MAPPINGS") and getattr(module, "NODE_CLASS_MAPPINGS") is not None:
|
|
NODE_CLASS_MAPPINGS.update(module.NODE_CLASS_MAPPINGS)
|
|
if hasattr(module, "NODE_DISPLAY_NAME_MAPPINGS") and getattr(module, "NODE_DISPLAY_NAME_MAPPINGS") is not None:
|
|
NODE_DISPLAY_NAME_MAPPINGS.update(module.NODE_DISPLAY_NAME_MAPPINGS)
|
|
else:
|
|
print(f"Skip {module_path} module for custom nodes due to the lack of NODE_CLASS_MAPPINGS.")
|
|
except Exception as e:
|
|
print(traceback.format_exc())
|
|
print(f"Cannot import {module_path} module for custom nodes:", e)
|
|
|
|
def load_custom_nodes():
|
|
node_paths = folder_paths.get_folder_paths("custom_nodes")
|
|
for custom_node_path in node_paths:
|
|
possible_modules = os.listdir(custom_node_path)
|
|
if "__pycache__" in possible_modules:
|
|
possible_modules.remove("__pycache__")
|
|
|
|
for possible_module in possible_modules:
|
|
module_path = os.path.join(custom_node_path, possible_module)
|
|
if os.path.isfile(module_path) and os.path.splitext(module_path)[1] != ".py": continue
|
|
load_custom_node(module_path)
|
|
|
|
def init_custom_nodes():
|
|
load_custom_nodes()
|
|
load_custom_node(os.path.join(os.path.join(os.path.dirname(os.path.realpath(__file__)), "comfy_extras"), "nodes_hypernetwork.py"))
|
|
load_custom_node(os.path.join(os.path.join(os.path.dirname(os.path.realpath(__file__)), "comfy_extras"), "nodes_upscale_model.py"))
|
|
load_custom_node(os.path.join(os.path.join(os.path.dirname(os.path.realpath(__file__)), "comfy_extras"), "nodes_post_processing.py"))
|
|
load_custom_node(os.path.join(os.path.join(os.path.dirname(os.path.realpath(__file__)), "comfy_extras"), "nodes_mask.py"))
|