mirror of
https://github.com/comfyanonymous/ComfyUI.git
synced 2025-07-14 03:16:59 +08:00
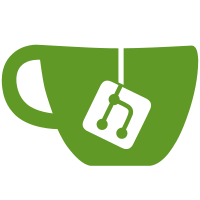
The created checkpoints contain workflow metadata that can be loaded by dragging them on top of the UI or loading them with the "Load" button. Checkpoints will be saved in fp16 or fp32 depending on the format ComfyUI is using for inference on your hardware. To force fp32 use: --force-fp32 Anything that patches the model weights like merging or loras will be saved. The output directory is currently set to: output/checkpoints but that might change in the future.
191 lines
8.2 KiB
Python
191 lines
8.2 KiB
Python
import torch
|
|
from comfy.ldm.modules.diffusionmodules.openaimodel import UNetModel
|
|
from comfy.ldm.modules.encoders.noise_aug_modules import CLIPEmbeddingNoiseAugmentation
|
|
from comfy.ldm.modules.diffusionmodules.util import make_beta_schedule
|
|
from comfy.ldm.modules.diffusionmodules.openaimodel import Timestep
|
|
import numpy as np
|
|
from . import utils
|
|
|
|
class BaseModel(torch.nn.Module):
|
|
def __init__(self, model_config, v_prediction=False):
|
|
super().__init__()
|
|
|
|
unet_config = model_config.unet_config
|
|
self.latent_format = model_config.latent_format
|
|
self.model_config = model_config
|
|
self.register_schedule(given_betas=None, beta_schedule="linear", timesteps=1000, linear_start=0.00085, linear_end=0.012, cosine_s=8e-3)
|
|
self.diffusion_model = UNetModel(**unet_config)
|
|
self.v_prediction = v_prediction
|
|
if self.v_prediction:
|
|
self.parameterization = "v"
|
|
else:
|
|
self.parameterization = "eps"
|
|
|
|
self.adm_channels = unet_config.get("adm_in_channels", None)
|
|
if self.adm_channels is None:
|
|
self.adm_channels = 0
|
|
print("v_prediction", v_prediction)
|
|
print("adm", self.adm_channels)
|
|
|
|
def register_schedule(self, given_betas=None, beta_schedule="linear", timesteps=1000,
|
|
linear_start=1e-4, linear_end=2e-2, cosine_s=8e-3):
|
|
if given_betas is not None:
|
|
betas = given_betas
|
|
else:
|
|
betas = make_beta_schedule(beta_schedule, timesteps, linear_start=linear_start, linear_end=linear_end, cosine_s=cosine_s)
|
|
alphas = 1. - betas
|
|
alphas_cumprod = np.cumprod(alphas, axis=0)
|
|
alphas_cumprod_prev = np.append(1., alphas_cumprod[:-1])
|
|
|
|
timesteps, = betas.shape
|
|
self.num_timesteps = int(timesteps)
|
|
self.linear_start = linear_start
|
|
self.linear_end = linear_end
|
|
|
|
self.register_buffer('betas', torch.tensor(betas, dtype=torch.float32))
|
|
self.register_buffer('alphas_cumprod', torch.tensor(alphas_cumprod, dtype=torch.float32))
|
|
self.register_buffer('alphas_cumprod_prev', torch.tensor(alphas_cumprod_prev, dtype=torch.float32))
|
|
|
|
def apply_model(self, x, t, c_concat=None, c_crossattn=None, c_adm=None, control=None, transformer_options={}):
|
|
if c_concat is not None:
|
|
xc = torch.cat([x] + c_concat, dim=1)
|
|
else:
|
|
xc = x
|
|
context = torch.cat(c_crossattn, 1)
|
|
return self.diffusion_model(xc, t, context=context, y=c_adm, control=control, transformer_options=transformer_options)
|
|
|
|
def get_dtype(self):
|
|
return self.diffusion_model.dtype
|
|
|
|
def is_adm(self):
|
|
return self.adm_channels > 0
|
|
|
|
def encode_adm(self, **kwargs):
|
|
return None
|
|
|
|
def load_model_weights(self, sd, unet_prefix=""):
|
|
to_load = {}
|
|
keys = list(sd.keys())
|
|
for k in keys:
|
|
if k.startswith(unet_prefix):
|
|
to_load[k[len(unet_prefix):]] = sd.pop(k)
|
|
|
|
m, u = self.diffusion_model.load_state_dict(to_load, strict=False)
|
|
if len(m) > 0:
|
|
print("unet missing:", m)
|
|
|
|
if len(u) > 0:
|
|
print("unet unexpected:", u)
|
|
del to_load
|
|
return self
|
|
|
|
def process_latent_in(self, latent):
|
|
return self.latent_format.process_in(latent)
|
|
|
|
def process_latent_out(self, latent):
|
|
return self.latent_format.process_out(latent)
|
|
|
|
def state_dict_for_saving(self, clip_state_dict, vae_state_dict):
|
|
clip_state_dict = self.model_config.process_clip_state_dict_for_saving(clip_state_dict)
|
|
unet_state_dict = self.diffusion_model.state_dict()
|
|
unet_state_dict = self.model_config.process_unet_state_dict_for_saving(unet_state_dict)
|
|
vae_state_dict = self.model_config.process_vae_state_dict_for_saving(vae_state_dict)
|
|
if self.get_dtype() == torch.float16:
|
|
clip_state_dict = utils.convert_sd_to(clip_state_dict, torch.float16)
|
|
vae_state_dict = utils.convert_sd_to(vae_state_dict, torch.float16)
|
|
return {**unet_state_dict, **vae_state_dict, **clip_state_dict}
|
|
|
|
|
|
class SD21UNCLIP(BaseModel):
|
|
def __init__(self, model_config, noise_aug_config, v_prediction=True):
|
|
super().__init__(model_config, v_prediction)
|
|
self.noise_augmentor = CLIPEmbeddingNoiseAugmentation(**noise_aug_config)
|
|
|
|
def encode_adm(self, **kwargs):
|
|
unclip_conditioning = kwargs.get("unclip_conditioning", None)
|
|
device = kwargs["device"]
|
|
|
|
if unclip_conditioning is not None:
|
|
adm_inputs = []
|
|
weights = []
|
|
noise_aug = []
|
|
for unclip_cond in unclip_conditioning:
|
|
adm_cond = unclip_cond["clip_vision_output"].image_embeds
|
|
weight = unclip_cond["strength"]
|
|
noise_augment = unclip_cond["noise_augmentation"]
|
|
noise_level = round((self.noise_augmentor.max_noise_level - 1) * noise_augment)
|
|
c_adm, noise_level_emb = self.noise_augmentor(adm_cond.to(device), noise_level=torch.tensor([noise_level], device=device))
|
|
adm_out = torch.cat((c_adm, noise_level_emb), 1) * weight
|
|
weights.append(weight)
|
|
noise_aug.append(noise_augment)
|
|
adm_inputs.append(adm_out)
|
|
|
|
if len(noise_aug) > 1:
|
|
adm_out = torch.stack(adm_inputs).sum(0)
|
|
#TODO: add a way to control this
|
|
noise_augment = 0.05
|
|
noise_level = round((self.noise_augmentor.max_noise_level - 1) * noise_augment)
|
|
c_adm, noise_level_emb = self.noise_augmentor(adm_out[:, :self.noise_augmentor.time_embed.dim], noise_level=torch.tensor([noise_level], device=device))
|
|
adm_out = torch.cat((c_adm, noise_level_emb), 1)
|
|
else:
|
|
adm_out = torch.zeros((1, self.adm_channels))
|
|
|
|
return adm_out
|
|
|
|
class SDInpaint(BaseModel):
|
|
def __init__(self, model_config, v_prediction=False):
|
|
super().__init__(model_config, v_prediction)
|
|
self.concat_keys = ("mask", "masked_image")
|
|
|
|
class SDXLRefiner(BaseModel):
|
|
def __init__(self, model_config, v_prediction=False):
|
|
super().__init__(model_config, v_prediction)
|
|
self.embedder = Timestep(256)
|
|
|
|
def encode_adm(self, **kwargs):
|
|
clip_pooled = kwargs["pooled_output"]
|
|
width = kwargs.get("width", 768)
|
|
height = kwargs.get("height", 768)
|
|
crop_w = kwargs.get("crop_w", 0)
|
|
crop_h = kwargs.get("crop_h", 0)
|
|
|
|
if kwargs.get("prompt_type", "") == "negative":
|
|
aesthetic_score = kwargs.get("aesthetic_score", 2.5)
|
|
else:
|
|
aesthetic_score = kwargs.get("aesthetic_score", 6)
|
|
|
|
print(clip_pooled.shape, width, height, crop_w, crop_h, aesthetic_score)
|
|
out = []
|
|
out.append(self.embedder(torch.Tensor([width])))
|
|
out.append(self.embedder(torch.Tensor([height])))
|
|
out.append(self.embedder(torch.Tensor([crop_w])))
|
|
out.append(self.embedder(torch.Tensor([crop_h])))
|
|
out.append(self.embedder(torch.Tensor([aesthetic_score])))
|
|
flat = torch.flatten(torch.cat(out))[None, ]
|
|
return torch.cat((clip_pooled.to(flat.device), flat), dim=1)
|
|
|
|
class SDXL(BaseModel):
|
|
def __init__(self, model_config, v_prediction=False):
|
|
super().__init__(model_config, v_prediction)
|
|
self.embedder = Timestep(256)
|
|
|
|
def encode_adm(self, **kwargs):
|
|
clip_pooled = kwargs["pooled_output"]
|
|
width = kwargs.get("width", 768)
|
|
height = kwargs.get("height", 768)
|
|
crop_w = kwargs.get("crop_w", 0)
|
|
crop_h = kwargs.get("crop_h", 0)
|
|
target_width = kwargs.get("target_width", width)
|
|
target_height = kwargs.get("target_height", height)
|
|
|
|
print(clip_pooled.shape, width, height, crop_w, crop_h, target_width, target_height)
|
|
out = []
|
|
out.append(self.embedder(torch.Tensor([width])))
|
|
out.append(self.embedder(torch.Tensor([height])))
|
|
out.append(self.embedder(torch.Tensor([crop_w])))
|
|
out.append(self.embedder(torch.Tensor([crop_h])))
|
|
out.append(self.embedder(torch.Tensor([target_width])))
|
|
out.append(self.embedder(torch.Tensor([target_height])))
|
|
flat = torch.flatten(torch.cat(out))[None, ]
|
|
return torch.cat((clip_pooled.to(flat.device), flat), dim=1)
|