mirror of
https://github.com/comfyanonymous/ComfyUI.git
synced 2025-01-25 15:55:18 +00:00
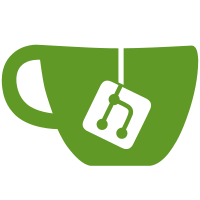
When generating images with fp8_e4_m3 Flux and batch size >1, using --fast, ComfyUI throws a "view size is not compatible with input tensor's size and stride" error pointing at the first of these two calls to view. As reshape is semantically equivalent to view except for working on a broader set of inputs, there should be no downside to changing this. The only difference is that it clones the underlying data in cases where .view would error out. I have confirmed that the output still looks as expected, but cannot confirm that no mutable use is made of the tensors anywhere. Note that --fast is only marginally faster than the default.
283 lines
10 KiB
Python
283 lines
10 KiB
Python
"""
|
|
This file is part of ComfyUI.
|
|
Copyright (C) 2024 Stability AI
|
|
|
|
This program is free software: you can redistribute it and/or modify
|
|
it under the terms of the GNU General Public License as published by
|
|
the Free Software Foundation, either version 3 of the License, or
|
|
(at your option) any later version.
|
|
|
|
This program is distributed in the hope that it will be useful,
|
|
but WITHOUT ANY WARRANTY; without even the implied warranty of
|
|
MERCHANTABILITY or FITNESS FOR A PARTICULAR PURPOSE. See the
|
|
GNU General Public License for more details.
|
|
|
|
You should have received a copy of the GNU General Public License
|
|
along with this program. If not, see <https://www.gnu.org/licenses/>.
|
|
"""
|
|
|
|
import torch
|
|
import comfy.model_management
|
|
from comfy.cli_args import args
|
|
|
|
def cast_to(weight, dtype=None, device=None, non_blocking=False):
|
|
if (dtype is None or weight.dtype == dtype) and (device is None or weight.device == device):
|
|
return weight
|
|
r = torch.empty_like(weight, dtype=dtype, device=device)
|
|
r.copy_(weight, non_blocking=non_blocking)
|
|
return r
|
|
|
|
def cast_to_input(weight, input, non_blocking=False):
|
|
return cast_to(weight, input.dtype, input.device, non_blocking=non_blocking)
|
|
|
|
def cast_bias_weight(s, input=None, dtype=None, device=None):
|
|
if input is not None:
|
|
if dtype is None:
|
|
dtype = input.dtype
|
|
if device is None:
|
|
device = input.device
|
|
|
|
bias = None
|
|
non_blocking = comfy.model_management.device_supports_non_blocking(device)
|
|
if s.bias is not None:
|
|
bias = cast_to(s.bias, dtype, device, non_blocking=non_blocking)
|
|
if s.bias_function is not None:
|
|
bias = s.bias_function(bias)
|
|
weight = cast_to(s.weight, dtype, device, non_blocking=non_blocking)
|
|
if s.weight_function is not None:
|
|
weight = s.weight_function(weight)
|
|
return weight, bias
|
|
|
|
class CastWeightBiasOp:
|
|
comfy_cast_weights = False
|
|
weight_function = None
|
|
bias_function = None
|
|
|
|
class disable_weight_init:
|
|
class Linear(torch.nn.Linear, CastWeightBiasOp):
|
|
def reset_parameters(self):
|
|
return None
|
|
|
|
def forward_comfy_cast_weights(self, input):
|
|
weight, bias = cast_bias_weight(self, input)
|
|
return torch.nn.functional.linear(input, weight, bias)
|
|
|
|
def forward(self, *args, **kwargs):
|
|
if self.comfy_cast_weights:
|
|
return self.forward_comfy_cast_weights(*args, **kwargs)
|
|
else:
|
|
return super().forward(*args, **kwargs)
|
|
|
|
class Conv1d(torch.nn.Conv1d, CastWeightBiasOp):
|
|
def reset_parameters(self):
|
|
return None
|
|
|
|
def forward_comfy_cast_weights(self, input):
|
|
weight, bias = cast_bias_weight(self, input)
|
|
return self._conv_forward(input, weight, bias)
|
|
|
|
def forward(self, *args, **kwargs):
|
|
if self.comfy_cast_weights:
|
|
return self.forward_comfy_cast_weights(*args, **kwargs)
|
|
else:
|
|
return super().forward(*args, **kwargs)
|
|
|
|
class Conv2d(torch.nn.Conv2d, CastWeightBiasOp):
|
|
def reset_parameters(self):
|
|
return None
|
|
|
|
def forward_comfy_cast_weights(self, input):
|
|
weight, bias = cast_bias_weight(self, input)
|
|
return self._conv_forward(input, weight, bias)
|
|
|
|
def forward(self, *args, **kwargs):
|
|
if self.comfy_cast_weights:
|
|
return self.forward_comfy_cast_weights(*args, **kwargs)
|
|
else:
|
|
return super().forward(*args, **kwargs)
|
|
|
|
class Conv3d(torch.nn.Conv3d, CastWeightBiasOp):
|
|
def reset_parameters(self):
|
|
return None
|
|
|
|
def forward_comfy_cast_weights(self, input):
|
|
weight, bias = cast_bias_weight(self, input)
|
|
return self._conv_forward(input, weight, bias)
|
|
|
|
def forward(self, *args, **kwargs):
|
|
if self.comfy_cast_weights:
|
|
return self.forward_comfy_cast_weights(*args, **kwargs)
|
|
else:
|
|
return super().forward(*args, **kwargs)
|
|
|
|
class GroupNorm(torch.nn.GroupNorm, CastWeightBiasOp):
|
|
def reset_parameters(self):
|
|
return None
|
|
|
|
def forward_comfy_cast_weights(self, input):
|
|
weight, bias = cast_bias_weight(self, input)
|
|
return torch.nn.functional.group_norm(input, self.num_groups, weight, bias, self.eps)
|
|
|
|
def forward(self, *args, **kwargs):
|
|
if self.comfy_cast_weights:
|
|
return self.forward_comfy_cast_weights(*args, **kwargs)
|
|
else:
|
|
return super().forward(*args, **kwargs)
|
|
|
|
|
|
class LayerNorm(torch.nn.LayerNorm, CastWeightBiasOp):
|
|
def reset_parameters(self):
|
|
return None
|
|
|
|
def forward_comfy_cast_weights(self, input):
|
|
if self.weight is not None:
|
|
weight, bias = cast_bias_weight(self, input)
|
|
else:
|
|
weight = None
|
|
bias = None
|
|
return torch.nn.functional.layer_norm(input, self.normalized_shape, weight, bias, self.eps)
|
|
|
|
def forward(self, *args, **kwargs):
|
|
if self.comfy_cast_weights:
|
|
return self.forward_comfy_cast_weights(*args, **kwargs)
|
|
else:
|
|
return super().forward(*args, **kwargs)
|
|
|
|
class ConvTranspose2d(torch.nn.ConvTranspose2d, CastWeightBiasOp):
|
|
def reset_parameters(self):
|
|
return None
|
|
|
|
def forward_comfy_cast_weights(self, input, output_size=None):
|
|
num_spatial_dims = 2
|
|
output_padding = self._output_padding(
|
|
input, output_size, self.stride, self.padding, self.kernel_size,
|
|
num_spatial_dims, self.dilation)
|
|
|
|
weight, bias = cast_bias_weight(self, input)
|
|
return torch.nn.functional.conv_transpose2d(
|
|
input, weight, bias, self.stride, self.padding,
|
|
output_padding, self.groups, self.dilation)
|
|
|
|
def forward(self, *args, **kwargs):
|
|
if self.comfy_cast_weights:
|
|
return self.forward_comfy_cast_weights(*args, **kwargs)
|
|
else:
|
|
return super().forward(*args, **kwargs)
|
|
|
|
class ConvTranspose1d(torch.nn.ConvTranspose1d, CastWeightBiasOp):
|
|
def reset_parameters(self):
|
|
return None
|
|
|
|
def forward_comfy_cast_weights(self, input, output_size=None):
|
|
num_spatial_dims = 1
|
|
output_padding = self._output_padding(
|
|
input, output_size, self.stride, self.padding, self.kernel_size,
|
|
num_spatial_dims, self.dilation)
|
|
|
|
weight, bias = cast_bias_weight(self, input)
|
|
return torch.nn.functional.conv_transpose1d(
|
|
input, weight, bias, self.stride, self.padding,
|
|
output_padding, self.groups, self.dilation)
|
|
|
|
def forward(self, *args, **kwargs):
|
|
if self.comfy_cast_weights:
|
|
return self.forward_comfy_cast_weights(*args, **kwargs)
|
|
else:
|
|
return super().forward(*args, **kwargs)
|
|
|
|
class Embedding(torch.nn.Embedding, CastWeightBiasOp):
|
|
def reset_parameters(self):
|
|
self.bias = None
|
|
return None
|
|
|
|
def forward_comfy_cast_weights(self, input, out_dtype=None):
|
|
output_dtype = out_dtype
|
|
if self.weight.dtype == torch.float16 or self.weight.dtype == torch.bfloat16:
|
|
out_dtype = None
|
|
weight, bias = cast_bias_weight(self, device=input.device, dtype=out_dtype)
|
|
return torch.nn.functional.embedding(input, weight, self.padding_idx, self.max_norm, self.norm_type, self.scale_grad_by_freq, self.sparse).to(dtype=output_dtype)
|
|
|
|
def forward(self, *args, **kwargs):
|
|
if self.comfy_cast_weights:
|
|
return self.forward_comfy_cast_weights(*args, **kwargs)
|
|
else:
|
|
if "out_dtype" in kwargs:
|
|
kwargs.pop("out_dtype")
|
|
return super().forward(*args, **kwargs)
|
|
|
|
@classmethod
|
|
def conv_nd(s, dims, *args, **kwargs):
|
|
if dims == 2:
|
|
return s.Conv2d(*args, **kwargs)
|
|
elif dims == 3:
|
|
return s.Conv3d(*args, **kwargs)
|
|
else:
|
|
raise ValueError(f"unsupported dimensions: {dims}")
|
|
|
|
|
|
class manual_cast(disable_weight_init):
|
|
class Linear(disable_weight_init.Linear):
|
|
comfy_cast_weights = True
|
|
|
|
class Conv1d(disable_weight_init.Conv1d):
|
|
comfy_cast_weights = True
|
|
|
|
class Conv2d(disable_weight_init.Conv2d):
|
|
comfy_cast_weights = True
|
|
|
|
class Conv3d(disable_weight_init.Conv3d):
|
|
comfy_cast_weights = True
|
|
|
|
class GroupNorm(disable_weight_init.GroupNorm):
|
|
comfy_cast_weights = True
|
|
|
|
class LayerNorm(disable_weight_init.LayerNorm):
|
|
comfy_cast_weights = True
|
|
|
|
class ConvTranspose2d(disable_weight_init.ConvTranspose2d):
|
|
comfy_cast_weights = True
|
|
|
|
class ConvTranspose1d(disable_weight_init.ConvTranspose1d):
|
|
comfy_cast_weights = True
|
|
|
|
class Embedding(disable_weight_init.Embedding):
|
|
comfy_cast_weights = True
|
|
|
|
|
|
def fp8_linear(self, input):
|
|
dtype = self.weight.dtype
|
|
if dtype not in [torch.float8_e4m3fn]:
|
|
return None
|
|
|
|
if len(input.shape) == 3:
|
|
inn = input.reshape(-1, input.shape[2]).to(dtype)
|
|
non_blocking = comfy.model_management.device_supports_non_blocking(input.device)
|
|
w = cast_to(self.weight, device=input.device, non_blocking=non_blocking).t()
|
|
|
|
if self.bias is not None:
|
|
o, _ = torch._scaled_mm(inn, w, out_dtype=input.dtype, bias=cast_to_input(self.bias, input, non_blocking=non_blocking))
|
|
else:
|
|
o, _ = torch._scaled_mm(inn, w, out_dtype=input.dtype)
|
|
|
|
return o.reshape((-1, input.shape[1], self.weight.shape[0]))
|
|
return None
|
|
|
|
class fp8_ops(manual_cast):
|
|
class Linear(manual_cast.Linear):
|
|
def forward_comfy_cast_weights(self, input):
|
|
out = fp8_linear(self, input)
|
|
if out is not None:
|
|
return out
|
|
|
|
weight, bias = cast_bias_weight(self, input)
|
|
return torch.nn.functional.linear(input, weight, bias)
|
|
|
|
|
|
def pick_operations(weight_dtype, compute_dtype, load_device=None):
|
|
if compute_dtype is None or weight_dtype == compute_dtype:
|
|
return disable_weight_init
|
|
if args.fast:
|
|
if comfy.model_management.supports_fp8_compute(load_device):
|
|
return fp8_ops
|
|
return manual_cast
|