mirror of
https://github.com/comfyanonymous/ComfyUI.git
synced 2025-01-12 02:45:16 +00:00
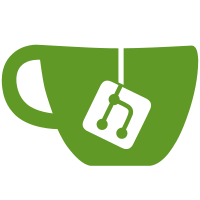
The created checkpoints contain workflow metadata that can be loaded by dragging them on top of the UI or loading them with the "Load" button. Checkpoints will be saved in fp16 or fp32 depending on the format ComfyUI is using for inference on your hardware. To force fp32 use: --force-fp32 Anything that patches the model weights like merging or loras will be saved. The output directory is currently set to: output/checkpoints but that might change in the future.
327 lines
10 KiB
JavaScript
327 lines
10 KiB
JavaScript
import { api } from "./api.js";
|
|
|
|
export function getPngMetadata(file) {
|
|
return new Promise((r) => {
|
|
const reader = new FileReader();
|
|
reader.onload = (event) => {
|
|
// Get the PNG data as a Uint8Array
|
|
const pngData = new Uint8Array(event.target.result);
|
|
const dataView = new DataView(pngData.buffer);
|
|
|
|
// Check that the PNG signature is present
|
|
if (dataView.getUint32(0) !== 0x89504e47) {
|
|
console.error("Not a valid PNG file");
|
|
r();
|
|
return;
|
|
}
|
|
|
|
// Start searching for chunks after the PNG signature
|
|
let offset = 8;
|
|
let txt_chunks = {};
|
|
// Loop through the chunks in the PNG file
|
|
while (offset < pngData.length) {
|
|
// Get the length of the chunk
|
|
const length = dataView.getUint32(offset);
|
|
// Get the chunk type
|
|
const type = String.fromCharCode(...pngData.slice(offset + 4, offset + 8));
|
|
if (type === "tEXt") {
|
|
// Get the keyword
|
|
let keyword_end = offset + 8;
|
|
while (pngData[keyword_end] !== 0) {
|
|
keyword_end++;
|
|
}
|
|
const keyword = String.fromCharCode(...pngData.slice(offset + 8, keyword_end));
|
|
// Get the text
|
|
const contentArraySegment = pngData.slice(keyword_end + 1, offset + 8 + length);
|
|
const contentJson = Array.from(contentArraySegment).map(s=>String.fromCharCode(s)).join('')
|
|
txt_chunks[keyword] = contentJson;
|
|
}
|
|
|
|
offset += 12 + length;
|
|
}
|
|
|
|
r(txt_chunks);
|
|
};
|
|
|
|
reader.readAsArrayBuffer(file);
|
|
});
|
|
}
|
|
|
|
export function getLatentMetadata(file) {
|
|
return new Promise((r) => {
|
|
const reader = new FileReader();
|
|
reader.onload = (event) => {
|
|
const safetensorsData = new Uint8Array(event.target.result);
|
|
const dataView = new DataView(safetensorsData.buffer);
|
|
let header_size = dataView.getUint32(0, true);
|
|
let offset = 8;
|
|
let header = JSON.parse(new TextDecoder().decode(safetensorsData.slice(offset, offset + header_size)));
|
|
r(header.__metadata__);
|
|
};
|
|
|
|
var slice = file.slice(0, 1024 * 1024 * 4);
|
|
reader.readAsArrayBuffer(slice);
|
|
});
|
|
}
|
|
|
|
export async function importA1111(graph, parameters) {
|
|
const p = parameters.lastIndexOf("\nSteps:");
|
|
if (p > -1) {
|
|
const embeddings = await api.getEmbeddings();
|
|
const opts = parameters
|
|
.substr(p)
|
|
.split("\n")[1]
|
|
.split(",")
|
|
.reduce((p, n) => {
|
|
const s = n.split(":");
|
|
p[s[0].trim().toLowerCase()] = s[1].trim();
|
|
return p;
|
|
}, {});
|
|
const p2 = parameters.lastIndexOf("\nNegative prompt:", p);
|
|
if (p2 > -1) {
|
|
let positive = parameters.substr(0, p2).trim();
|
|
let negative = parameters.substring(p2 + 18, p).trim();
|
|
|
|
const ckptNode = LiteGraph.createNode("CheckpointLoaderSimple");
|
|
const clipSkipNode = LiteGraph.createNode("CLIPSetLastLayer");
|
|
const positiveNode = LiteGraph.createNode("CLIPTextEncode");
|
|
const negativeNode = LiteGraph.createNode("CLIPTextEncode");
|
|
const samplerNode = LiteGraph.createNode("KSampler");
|
|
const imageNode = LiteGraph.createNode("EmptyLatentImage");
|
|
const vaeNode = LiteGraph.createNode("VAEDecode");
|
|
const vaeLoaderNode = LiteGraph.createNode("VAELoader");
|
|
const saveNode = LiteGraph.createNode("SaveImage");
|
|
let hrSamplerNode = null;
|
|
|
|
const ceil64 = (v) => Math.ceil(v / 64) * 64;
|
|
|
|
function getWidget(node, name) {
|
|
return node.widgets.find((w) => w.name === name);
|
|
}
|
|
|
|
function setWidgetValue(node, name, value, isOptionPrefix) {
|
|
const w = getWidget(node, name);
|
|
if (isOptionPrefix) {
|
|
const o = w.options.values.find((w) => w.startsWith(value));
|
|
if (o) {
|
|
w.value = o;
|
|
} else {
|
|
console.warn(`Unknown value '${value}' for widget '${name}'`, node);
|
|
w.value = value;
|
|
}
|
|
} else {
|
|
w.value = value;
|
|
}
|
|
}
|
|
|
|
function createLoraNodes(clipNode, text, prevClip, prevModel) {
|
|
const loras = [];
|
|
text = text.replace(/<lora:([^:]+:[^>]+)>/g, function (m, c) {
|
|
const s = c.split(":");
|
|
const weight = parseFloat(s[1]);
|
|
if (isNaN(weight)) {
|
|
console.warn("Invalid LORA", m);
|
|
} else {
|
|
loras.push({ name: s[0], weight });
|
|
}
|
|
return "";
|
|
});
|
|
|
|
for (const l of loras) {
|
|
const loraNode = LiteGraph.createNode("LoraLoader");
|
|
graph.add(loraNode);
|
|
setWidgetValue(loraNode, "lora_name", l.name, true);
|
|
setWidgetValue(loraNode, "strength_model", l.weight);
|
|
setWidgetValue(loraNode, "strength_clip", l.weight);
|
|
prevModel.node.connect(prevModel.index, loraNode, 0);
|
|
prevClip.node.connect(prevClip.index, loraNode, 1);
|
|
prevModel = { node: loraNode, index: 0 };
|
|
prevClip = { node: loraNode, index: 1 };
|
|
}
|
|
|
|
prevClip.node.connect(1, clipNode, 0);
|
|
prevModel.node.connect(0, samplerNode, 0);
|
|
if (hrSamplerNode) {
|
|
prevModel.node.connect(0, hrSamplerNode, 0);
|
|
}
|
|
|
|
return { text, prevModel, prevClip };
|
|
}
|
|
|
|
function replaceEmbeddings(text) {
|
|
if(!embeddings.length) return text;
|
|
return text.replaceAll(
|
|
new RegExp(
|
|
"\\b(" + embeddings.map((e) => e.replace(/[.*+?^${}()|[\]\\]/g, "\\$&")).join("\\b|\\b") + ")\\b",
|
|
"ig"
|
|
),
|
|
"embedding:$1"
|
|
);
|
|
}
|
|
|
|
function popOpt(name) {
|
|
const v = opts[name];
|
|
delete opts[name];
|
|
return v;
|
|
}
|
|
|
|
graph.clear();
|
|
graph.add(ckptNode);
|
|
graph.add(clipSkipNode);
|
|
graph.add(positiveNode);
|
|
graph.add(negativeNode);
|
|
graph.add(samplerNode);
|
|
graph.add(imageNode);
|
|
graph.add(vaeNode);
|
|
graph.add(vaeLoaderNode);
|
|
graph.add(saveNode);
|
|
|
|
ckptNode.connect(1, clipSkipNode, 0);
|
|
clipSkipNode.connect(0, positiveNode, 0);
|
|
clipSkipNode.connect(0, negativeNode, 0);
|
|
ckptNode.connect(0, samplerNode, 0);
|
|
positiveNode.connect(0, samplerNode, 1);
|
|
negativeNode.connect(0, samplerNode, 2);
|
|
imageNode.connect(0, samplerNode, 3);
|
|
vaeNode.connect(0, saveNode, 0);
|
|
samplerNode.connect(0, vaeNode, 0);
|
|
vaeLoaderNode.connect(0, vaeNode, 1);
|
|
|
|
const handlers = {
|
|
model(v) {
|
|
setWidgetValue(ckptNode, "ckpt_name", v, true);
|
|
},
|
|
"cfg scale"(v) {
|
|
setWidgetValue(samplerNode, "cfg", +v);
|
|
},
|
|
"clip skip"(v) {
|
|
setWidgetValue(clipSkipNode, "stop_at_clip_layer", -v);
|
|
},
|
|
sampler(v) {
|
|
let name = v.toLowerCase().replace("++", "pp").replaceAll(" ", "_");
|
|
if (name.includes("karras")) {
|
|
name = name.replace("karras", "").replace(/_+$/, "");
|
|
setWidgetValue(samplerNode, "scheduler", "karras");
|
|
} else {
|
|
setWidgetValue(samplerNode, "scheduler", "normal");
|
|
}
|
|
const w = getWidget(samplerNode, "sampler_name");
|
|
const o = w.options.values.find((w) => w === name || w === "sample_" + name);
|
|
if (o) {
|
|
setWidgetValue(samplerNode, "sampler_name", o);
|
|
}
|
|
},
|
|
size(v) {
|
|
const wxh = v.split("x");
|
|
const w = ceil64(+wxh[0]);
|
|
const h = ceil64(+wxh[1]);
|
|
const hrUp = popOpt("hires upscale");
|
|
const hrSz = popOpt("hires resize");
|
|
let hrMethod = popOpt("hires upscaler");
|
|
|
|
setWidgetValue(imageNode, "width", w);
|
|
setWidgetValue(imageNode, "height", h);
|
|
|
|
if (hrUp || hrSz) {
|
|
let uw, uh;
|
|
if (hrUp) {
|
|
uw = w * hrUp;
|
|
uh = h * hrUp;
|
|
} else {
|
|
const s = hrSz.split("x");
|
|
uw = +s[0];
|
|
uh = +s[1];
|
|
}
|
|
|
|
let upscaleNode;
|
|
let latentNode;
|
|
|
|
if (hrMethod.startsWith("Latent")) {
|
|
latentNode = upscaleNode = LiteGraph.createNode("LatentUpscale");
|
|
graph.add(upscaleNode);
|
|
samplerNode.connect(0, upscaleNode, 0);
|
|
|
|
switch (hrMethod) {
|
|
case "Latent (nearest-exact)":
|
|
hrMethod = "nearest-exact";
|
|
break;
|
|
}
|
|
setWidgetValue(upscaleNode, "upscale_method", hrMethod, true);
|
|
} else {
|
|
const decode = LiteGraph.createNode("VAEDecodeTiled");
|
|
graph.add(decode);
|
|
samplerNode.connect(0, decode, 0);
|
|
vaeLoaderNode.connect(0, decode, 1);
|
|
|
|
const upscaleLoaderNode = LiteGraph.createNode("UpscaleModelLoader");
|
|
graph.add(upscaleLoaderNode);
|
|
setWidgetValue(upscaleLoaderNode, "model_name", hrMethod, true);
|
|
|
|
const modelUpscaleNode = LiteGraph.createNode("ImageUpscaleWithModel");
|
|
graph.add(modelUpscaleNode);
|
|
decode.connect(0, modelUpscaleNode, 1);
|
|
upscaleLoaderNode.connect(0, modelUpscaleNode, 0);
|
|
|
|
upscaleNode = LiteGraph.createNode("ImageScale");
|
|
graph.add(upscaleNode);
|
|
modelUpscaleNode.connect(0, upscaleNode, 0);
|
|
|
|
const vaeEncodeNode = (latentNode = LiteGraph.createNode("VAEEncodeTiled"));
|
|
graph.add(vaeEncodeNode);
|
|
upscaleNode.connect(0, vaeEncodeNode, 0);
|
|
vaeLoaderNode.connect(0, vaeEncodeNode, 1);
|
|
}
|
|
|
|
setWidgetValue(upscaleNode, "width", ceil64(uw));
|
|
setWidgetValue(upscaleNode, "height", ceil64(uh));
|
|
|
|
hrSamplerNode = LiteGraph.createNode("KSampler");
|
|
graph.add(hrSamplerNode);
|
|
ckptNode.connect(0, hrSamplerNode, 0);
|
|
positiveNode.connect(0, hrSamplerNode, 1);
|
|
negativeNode.connect(0, hrSamplerNode, 2);
|
|
latentNode.connect(0, hrSamplerNode, 3);
|
|
hrSamplerNode.connect(0, vaeNode, 0);
|
|
}
|
|
},
|
|
steps(v) {
|
|
setWidgetValue(samplerNode, "steps", +v);
|
|
},
|
|
seed(v) {
|
|
setWidgetValue(samplerNode, "seed", +v);
|
|
},
|
|
};
|
|
|
|
for (const opt in opts) {
|
|
if (opt in handlers) {
|
|
handlers[opt](popOpt(opt));
|
|
}
|
|
}
|
|
|
|
if (hrSamplerNode) {
|
|
setWidgetValue(hrSamplerNode, "steps", getWidget(samplerNode, "steps").value);
|
|
setWidgetValue(hrSamplerNode, "cfg", getWidget(samplerNode, "cfg").value);
|
|
setWidgetValue(hrSamplerNode, "scheduler", getWidget(samplerNode, "scheduler").value);
|
|
setWidgetValue(hrSamplerNode, "sampler_name", getWidget(samplerNode, "sampler_name").value);
|
|
setWidgetValue(hrSamplerNode, "denoise", +(popOpt("denoising strength") || "1"));
|
|
}
|
|
|
|
let n = createLoraNodes(positiveNode, positive, { node: clipSkipNode, index: 0 }, { node: ckptNode, index: 0 });
|
|
positive = n.text;
|
|
n = createLoraNodes(negativeNode, negative, n.prevClip, n.prevModel);
|
|
negative = n.text;
|
|
|
|
setWidgetValue(positiveNode, "text", replaceEmbeddings(positive));
|
|
setWidgetValue(negativeNode, "text", replaceEmbeddings(negative));
|
|
|
|
graph.arrange();
|
|
|
|
for (const opt of ["model hash", "ensd"]) {
|
|
delete opts[opt];
|
|
}
|
|
|
|
console.warn("Unhandled parameters:", opts);
|
|
}
|
|
}
|
|
}
|