mirror of
https://github.com/comfyanonymous/ComfyUI.git
synced 2025-07-09 08:47:17 +08:00
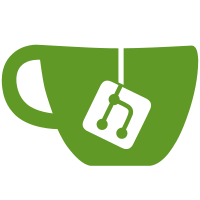
Use the "lcm" sampler to sample them, you also have to use the ModelSamplingDiscrete node to set them as lcm models to use them properly.
129 lines
4.3 KiB
Python
129 lines
4.3 KiB
Python
import folder_paths
|
|
import comfy.sd
|
|
import comfy.model_sampling
|
|
import torch
|
|
|
|
class LCM(comfy.model_sampling.EPS):
|
|
def calculate_denoised(self, sigma, model_output, model_input):
|
|
timestep = self.timestep(sigma).view(sigma.shape[:1] + (1,) * (model_output.ndim - 1))
|
|
sigma = sigma.view(sigma.shape[:1] + (1,) * (model_output.ndim - 1))
|
|
x0 = model_input - model_output * sigma
|
|
|
|
sigma_data = 0.5
|
|
scaled_timestep = timestep * 10.0 #timestep_scaling
|
|
|
|
c_skip = sigma_data**2 / (scaled_timestep**2 + sigma_data**2)
|
|
c_out = scaled_timestep / (scaled_timestep**2 + sigma_data**2) ** 0.5
|
|
|
|
return c_out * x0 + c_skip * model_input
|
|
|
|
class ModelSamplingDiscreteLCM(torch.nn.Module):
|
|
def __init__(self):
|
|
super().__init__()
|
|
self.sigma_data = 1.0
|
|
timesteps = 1000
|
|
beta_start = 0.00085
|
|
beta_end = 0.012
|
|
|
|
betas = torch.linspace(beta_start**0.5, beta_end**0.5, timesteps, dtype=torch.float32) ** 2
|
|
alphas = 1.0 - betas
|
|
alphas_cumprod = torch.cumprod(alphas, dim=0)
|
|
|
|
original_timesteps = 50
|
|
self.skip_steps = timesteps // original_timesteps
|
|
|
|
|
|
alphas_cumprod_valid = torch.zeros((original_timesteps), dtype=torch.float32)
|
|
for x in range(original_timesteps):
|
|
alphas_cumprod_valid[original_timesteps - 1 - x] = alphas_cumprod[timesteps - 1 - x * self.skip_steps]
|
|
|
|
sigmas = ((1 - alphas_cumprod_valid) / alphas_cumprod_valid) ** 0.5
|
|
self.set_sigmas(sigmas)
|
|
|
|
def set_sigmas(self, sigmas):
|
|
self.register_buffer('sigmas', sigmas)
|
|
self.register_buffer('log_sigmas', sigmas.log())
|
|
|
|
@property
|
|
def sigma_min(self):
|
|
return self.sigmas[0]
|
|
|
|
@property
|
|
def sigma_max(self):
|
|
return self.sigmas[-1]
|
|
|
|
def timestep(self, sigma):
|
|
log_sigma = sigma.log()
|
|
dists = log_sigma.to(self.log_sigmas.device) - self.log_sigmas[:, None]
|
|
return dists.abs().argmin(dim=0).view(sigma.shape) * self.skip_steps + (self.skip_steps - 1)
|
|
|
|
def sigma(self, timestep):
|
|
t = torch.clamp(((timestep - (self.skip_steps - 1)) / self.skip_steps).float(), min=0, max=(len(self.sigmas) - 1))
|
|
low_idx = t.floor().long()
|
|
high_idx = t.ceil().long()
|
|
w = t.frac()
|
|
log_sigma = (1 - w) * self.log_sigmas[low_idx] + w * self.log_sigmas[high_idx]
|
|
return log_sigma.exp()
|
|
|
|
def percent_to_sigma(self, percent):
|
|
return self.sigma(torch.tensor(percent * 999.0))
|
|
|
|
|
|
def rescale_zero_terminal_snr_sigmas(sigmas):
|
|
alphas_cumprod = 1 / ((sigmas * sigmas) + 1)
|
|
alphas_bar_sqrt = alphas_cumprod.sqrt()
|
|
|
|
# Store old values.
|
|
alphas_bar_sqrt_0 = alphas_bar_sqrt[0].clone()
|
|
alphas_bar_sqrt_T = alphas_bar_sqrt[-1].clone()
|
|
|
|
# Shift so the last timestep is zero.
|
|
alphas_bar_sqrt -= (alphas_bar_sqrt_T)
|
|
|
|
# Scale so the first timestep is back to the old value.
|
|
alphas_bar_sqrt *= alphas_bar_sqrt_0 / (alphas_bar_sqrt_0 - alphas_bar_sqrt_T)
|
|
|
|
# Convert alphas_bar_sqrt to betas
|
|
alphas_bar = alphas_bar_sqrt**2 # Revert sqrt
|
|
alphas_bar[-1] = 4.8973451890853435e-08
|
|
return ((1 - alphas_bar) / alphas_bar) ** 0.5
|
|
|
|
class ModelSamplingDiscrete:
|
|
@classmethod
|
|
def INPUT_TYPES(s):
|
|
return {"required": { "model": ("MODEL",),
|
|
"sampling": (["eps", "v_prediction", "lcm"],),
|
|
"zsnr": ("BOOLEAN", {"default": False}),
|
|
}}
|
|
|
|
RETURN_TYPES = ("MODEL",)
|
|
FUNCTION = "patch"
|
|
|
|
CATEGORY = "advanced/model"
|
|
|
|
def patch(self, model, sampling, zsnr):
|
|
m = model.clone()
|
|
|
|
sampling_base = comfy.model_sampling.ModelSamplingDiscrete
|
|
if sampling == "eps":
|
|
sampling_type = comfy.model_sampling.EPS
|
|
elif sampling == "v_prediction":
|
|
sampling_type = comfy.model_sampling.V_PREDICTION
|
|
elif sampling == "lcm":
|
|
sampling_type = LCM
|
|
sampling_base = ModelSamplingDiscreteLCM
|
|
|
|
class ModelSamplingAdvanced(sampling_base, sampling_type):
|
|
pass
|
|
|
|
model_sampling = ModelSamplingAdvanced()
|
|
if zsnr:
|
|
model_sampling.set_sigmas(rescale_zero_terminal_snr_sigmas(model_sampling.sigmas))
|
|
|
|
m.add_object_patch("model_sampling", model_sampling)
|
|
return (m, )
|
|
|
|
NODE_CLASS_MAPPINGS = {
|
|
"ModelSamplingDiscrete": ModelSamplingDiscrete,
|
|
}
|