mirror of
https://github.com/comfyanonymous/ComfyUI.git
synced 2025-01-25 15:55:18 +00:00
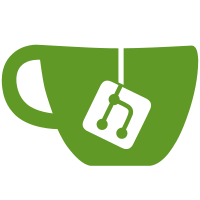
This is the code to load the model and inference it with only a text prompt. This commit does not contain the nodes to properly use it with an image input. This supports both the original SD1 instructpix2pix model and the diffusers SDXL one.
477 lines
16 KiB
Python
477 lines
16 KiB
Python
import torch
|
|
from . import model_base
|
|
from . import utils
|
|
|
|
from . import sd1_clip
|
|
from . import sd2_clip
|
|
from . import sdxl_clip
|
|
|
|
from . import supported_models_base
|
|
from . import latent_formats
|
|
|
|
from . import diffusers_convert
|
|
|
|
class SD15(supported_models_base.BASE):
|
|
unet_config = {
|
|
"context_dim": 768,
|
|
"model_channels": 320,
|
|
"use_linear_in_transformer": False,
|
|
"adm_in_channels": None,
|
|
"use_temporal_attention": False,
|
|
}
|
|
|
|
unet_extra_config = {
|
|
"num_heads": 8,
|
|
"num_head_channels": -1,
|
|
}
|
|
|
|
latent_format = latent_formats.SD15
|
|
|
|
def process_clip_state_dict(self, state_dict):
|
|
k = list(state_dict.keys())
|
|
for x in k:
|
|
if x.startswith("cond_stage_model.transformer.") and not x.startswith("cond_stage_model.transformer.text_model."):
|
|
y = x.replace("cond_stage_model.transformer.", "cond_stage_model.transformer.text_model.")
|
|
state_dict[y] = state_dict.pop(x)
|
|
|
|
if 'cond_stage_model.transformer.text_model.embeddings.position_ids' in state_dict:
|
|
ids = state_dict['cond_stage_model.transformer.text_model.embeddings.position_ids']
|
|
if ids.dtype == torch.float32:
|
|
state_dict['cond_stage_model.transformer.text_model.embeddings.position_ids'] = ids.round()
|
|
|
|
replace_prefix = {}
|
|
replace_prefix["cond_stage_model."] = "clip_l."
|
|
state_dict = utils.state_dict_prefix_replace(state_dict, replace_prefix, filter_keys=True)
|
|
return state_dict
|
|
|
|
def process_clip_state_dict_for_saving(self, state_dict):
|
|
pop_keys = ["clip_l.transformer.text_projection.weight", "clip_l.logit_scale"]
|
|
for p in pop_keys:
|
|
if p in state_dict:
|
|
state_dict.pop(p)
|
|
|
|
replace_prefix = {"clip_l.": "cond_stage_model."}
|
|
return utils.state_dict_prefix_replace(state_dict, replace_prefix)
|
|
|
|
def clip_target(self):
|
|
return supported_models_base.ClipTarget(sd1_clip.SD1Tokenizer, sd1_clip.SD1ClipModel)
|
|
|
|
class SD20(supported_models_base.BASE):
|
|
unet_config = {
|
|
"context_dim": 1024,
|
|
"model_channels": 320,
|
|
"use_linear_in_transformer": True,
|
|
"adm_in_channels": None,
|
|
"use_temporal_attention": False,
|
|
}
|
|
|
|
latent_format = latent_formats.SD15
|
|
|
|
def model_type(self, state_dict, prefix=""):
|
|
if self.unet_config["in_channels"] == 4: #SD2.0 inpainting models are not v prediction
|
|
k = "{}output_blocks.11.1.transformer_blocks.0.norm1.bias".format(prefix)
|
|
out = state_dict.get(k, None)
|
|
if out is not None and torch.std(out, unbiased=False) > 0.09: # not sure how well this will actually work. I guess we will find out.
|
|
return model_base.ModelType.V_PREDICTION
|
|
return model_base.ModelType.EPS
|
|
|
|
def process_clip_state_dict(self, state_dict):
|
|
replace_prefix = {}
|
|
replace_prefix["conditioner.embedders.0.model."] = "clip_h." #SD2 in sgm format
|
|
replace_prefix["cond_stage_model.model."] = "clip_h."
|
|
state_dict = utils.state_dict_prefix_replace(state_dict, replace_prefix, filter_keys=True)
|
|
state_dict = utils.clip_text_transformers_convert(state_dict, "clip_h.", "clip_h.transformer.")
|
|
return state_dict
|
|
|
|
def process_clip_state_dict_for_saving(self, state_dict):
|
|
replace_prefix = {}
|
|
replace_prefix["clip_h"] = "cond_stage_model.model"
|
|
state_dict = utils.state_dict_prefix_replace(state_dict, replace_prefix)
|
|
state_dict = diffusers_convert.convert_text_enc_state_dict_v20(state_dict)
|
|
return state_dict
|
|
|
|
def clip_target(self):
|
|
return supported_models_base.ClipTarget(sd2_clip.SD2Tokenizer, sd2_clip.SD2ClipModel)
|
|
|
|
class SD21UnclipL(SD20):
|
|
unet_config = {
|
|
"context_dim": 1024,
|
|
"model_channels": 320,
|
|
"use_linear_in_transformer": True,
|
|
"adm_in_channels": 1536,
|
|
"use_temporal_attention": False,
|
|
}
|
|
|
|
clip_vision_prefix = "embedder.model.visual."
|
|
noise_aug_config = {"noise_schedule_config": {"timesteps": 1000, "beta_schedule": "squaredcos_cap_v2"}, "timestep_dim": 768}
|
|
|
|
|
|
class SD21UnclipH(SD20):
|
|
unet_config = {
|
|
"context_dim": 1024,
|
|
"model_channels": 320,
|
|
"use_linear_in_transformer": True,
|
|
"adm_in_channels": 2048,
|
|
"use_temporal_attention": False,
|
|
}
|
|
|
|
clip_vision_prefix = "embedder.model.visual."
|
|
noise_aug_config = {"noise_schedule_config": {"timesteps": 1000, "beta_schedule": "squaredcos_cap_v2"}, "timestep_dim": 1024}
|
|
|
|
class SDXLRefiner(supported_models_base.BASE):
|
|
unet_config = {
|
|
"model_channels": 384,
|
|
"use_linear_in_transformer": True,
|
|
"context_dim": 1280,
|
|
"adm_in_channels": 2560,
|
|
"transformer_depth": [0, 0, 4, 4, 4, 4, 0, 0],
|
|
"use_temporal_attention": False,
|
|
}
|
|
|
|
latent_format = latent_formats.SDXL
|
|
|
|
def get_model(self, state_dict, prefix="", device=None):
|
|
return model_base.SDXLRefiner(self, device=device)
|
|
|
|
def process_clip_state_dict(self, state_dict):
|
|
keys_to_replace = {}
|
|
replace_prefix = {}
|
|
replace_prefix["conditioner.embedders.0.model."] = "clip_g."
|
|
state_dict = utils.state_dict_prefix_replace(state_dict, replace_prefix, filter_keys=True)
|
|
|
|
state_dict = utils.clip_text_transformers_convert(state_dict, "clip_g.", "clip_g.transformer.")
|
|
state_dict = utils.state_dict_key_replace(state_dict, keys_to_replace)
|
|
return state_dict
|
|
|
|
def process_clip_state_dict_for_saving(self, state_dict):
|
|
replace_prefix = {}
|
|
state_dict_g = diffusers_convert.convert_text_enc_state_dict_v20(state_dict, "clip_g")
|
|
if "clip_g.transformer.text_model.embeddings.position_ids" in state_dict_g:
|
|
state_dict_g.pop("clip_g.transformer.text_model.embeddings.position_ids")
|
|
replace_prefix["clip_g"] = "conditioner.embedders.0.model"
|
|
state_dict_g = utils.state_dict_prefix_replace(state_dict_g, replace_prefix)
|
|
return state_dict_g
|
|
|
|
def clip_target(self):
|
|
return supported_models_base.ClipTarget(sdxl_clip.SDXLTokenizer, sdxl_clip.SDXLRefinerClipModel)
|
|
|
|
class SDXL(supported_models_base.BASE):
|
|
unet_config = {
|
|
"model_channels": 320,
|
|
"use_linear_in_transformer": True,
|
|
"transformer_depth": [0, 0, 2, 2, 10, 10],
|
|
"context_dim": 2048,
|
|
"adm_in_channels": 2816,
|
|
"use_temporal_attention": False,
|
|
}
|
|
|
|
latent_format = latent_formats.SDXL
|
|
|
|
def model_type(self, state_dict, prefix=""):
|
|
if 'edm_mean' in state_dict and 'edm_std' in state_dict: #Playground V2.5
|
|
self.latent_format = latent_formats.SDXL_Playground_2_5()
|
|
self.sampling_settings["sigma_data"] = 0.5
|
|
self.sampling_settings["sigma_max"] = 80.0
|
|
self.sampling_settings["sigma_min"] = 0.002
|
|
return model_base.ModelType.EDM
|
|
elif "v_pred" in state_dict:
|
|
return model_base.ModelType.V_PREDICTION
|
|
else:
|
|
return model_base.ModelType.EPS
|
|
|
|
def get_model(self, state_dict, prefix="", device=None):
|
|
out = model_base.SDXL(self, model_type=self.model_type(state_dict, prefix), device=device)
|
|
if self.inpaint_model():
|
|
out.set_inpaint()
|
|
return out
|
|
|
|
def process_clip_state_dict(self, state_dict):
|
|
keys_to_replace = {}
|
|
replace_prefix = {}
|
|
|
|
replace_prefix["conditioner.embedders.0.transformer.text_model"] = "clip_l.transformer.text_model"
|
|
replace_prefix["conditioner.embedders.1.model."] = "clip_g."
|
|
state_dict = utils.state_dict_prefix_replace(state_dict, replace_prefix, filter_keys=True)
|
|
|
|
state_dict = utils.state_dict_key_replace(state_dict, keys_to_replace)
|
|
state_dict = utils.clip_text_transformers_convert(state_dict, "clip_g.", "clip_g.transformer.")
|
|
return state_dict
|
|
|
|
def process_clip_state_dict_for_saving(self, state_dict):
|
|
replace_prefix = {}
|
|
keys_to_replace = {}
|
|
state_dict_g = diffusers_convert.convert_text_enc_state_dict_v20(state_dict, "clip_g")
|
|
for k in state_dict:
|
|
if k.startswith("clip_l"):
|
|
state_dict_g[k] = state_dict[k]
|
|
|
|
state_dict_g["clip_l.transformer.text_model.embeddings.position_ids"] = torch.arange(77).expand((1, -1))
|
|
pop_keys = ["clip_l.transformer.text_projection.weight", "clip_l.logit_scale"]
|
|
for p in pop_keys:
|
|
if p in state_dict_g:
|
|
state_dict_g.pop(p)
|
|
|
|
replace_prefix["clip_g"] = "conditioner.embedders.1.model"
|
|
replace_prefix["clip_l"] = "conditioner.embedders.0"
|
|
state_dict_g = utils.state_dict_prefix_replace(state_dict_g, replace_prefix)
|
|
return state_dict_g
|
|
|
|
def clip_target(self):
|
|
return supported_models_base.ClipTarget(sdxl_clip.SDXLTokenizer, sdxl_clip.SDXLClipModel)
|
|
|
|
class SSD1B(SDXL):
|
|
unet_config = {
|
|
"model_channels": 320,
|
|
"use_linear_in_transformer": True,
|
|
"transformer_depth": [0, 0, 2, 2, 4, 4],
|
|
"context_dim": 2048,
|
|
"adm_in_channels": 2816,
|
|
"use_temporal_attention": False,
|
|
}
|
|
|
|
class Segmind_Vega(SDXL):
|
|
unet_config = {
|
|
"model_channels": 320,
|
|
"use_linear_in_transformer": True,
|
|
"transformer_depth": [0, 0, 1, 1, 2, 2],
|
|
"context_dim": 2048,
|
|
"adm_in_channels": 2816,
|
|
"use_temporal_attention": False,
|
|
}
|
|
|
|
class KOALA_700M(SDXL):
|
|
unet_config = {
|
|
"model_channels": 320,
|
|
"use_linear_in_transformer": True,
|
|
"transformer_depth": [0, 2, 5],
|
|
"context_dim": 2048,
|
|
"adm_in_channels": 2816,
|
|
"use_temporal_attention": False,
|
|
}
|
|
|
|
class KOALA_1B(SDXL):
|
|
unet_config = {
|
|
"model_channels": 320,
|
|
"use_linear_in_transformer": True,
|
|
"transformer_depth": [0, 2, 6],
|
|
"context_dim": 2048,
|
|
"adm_in_channels": 2816,
|
|
"use_temporal_attention": False,
|
|
}
|
|
|
|
class SVD_img2vid(supported_models_base.BASE):
|
|
unet_config = {
|
|
"model_channels": 320,
|
|
"in_channels": 8,
|
|
"use_linear_in_transformer": True,
|
|
"transformer_depth": [1, 1, 1, 1, 1, 1, 0, 0],
|
|
"context_dim": 1024,
|
|
"adm_in_channels": 768,
|
|
"use_temporal_attention": True,
|
|
"use_temporal_resblock": True
|
|
}
|
|
|
|
clip_vision_prefix = "conditioner.embedders.0.open_clip.model.visual."
|
|
|
|
latent_format = latent_formats.SD15
|
|
|
|
sampling_settings = {"sigma_max": 700.0, "sigma_min": 0.002}
|
|
|
|
def get_model(self, state_dict, prefix="", device=None):
|
|
out = model_base.SVD_img2vid(self, device=device)
|
|
return out
|
|
|
|
def clip_target(self):
|
|
return None
|
|
|
|
class SV3D_u(SVD_img2vid):
|
|
unet_config = {
|
|
"model_channels": 320,
|
|
"in_channels": 8,
|
|
"use_linear_in_transformer": True,
|
|
"transformer_depth": [1, 1, 1, 1, 1, 1, 0, 0],
|
|
"context_dim": 1024,
|
|
"adm_in_channels": 256,
|
|
"use_temporal_attention": True,
|
|
"use_temporal_resblock": True
|
|
}
|
|
|
|
vae_key_prefix = ["conditioner.embedders.1.encoder."]
|
|
|
|
def get_model(self, state_dict, prefix="", device=None):
|
|
out = model_base.SV3D_u(self, device=device)
|
|
return out
|
|
|
|
class SV3D_p(SV3D_u):
|
|
unet_config = {
|
|
"model_channels": 320,
|
|
"in_channels": 8,
|
|
"use_linear_in_transformer": True,
|
|
"transformer_depth": [1, 1, 1, 1, 1, 1, 0, 0],
|
|
"context_dim": 1024,
|
|
"adm_in_channels": 1280,
|
|
"use_temporal_attention": True,
|
|
"use_temporal_resblock": True
|
|
}
|
|
|
|
|
|
def get_model(self, state_dict, prefix="", device=None):
|
|
out = model_base.SV3D_p(self, device=device)
|
|
return out
|
|
|
|
class Stable_Zero123(supported_models_base.BASE):
|
|
unet_config = {
|
|
"context_dim": 768,
|
|
"model_channels": 320,
|
|
"use_linear_in_transformer": False,
|
|
"adm_in_channels": None,
|
|
"use_temporal_attention": False,
|
|
"in_channels": 8,
|
|
}
|
|
|
|
unet_extra_config = {
|
|
"num_heads": 8,
|
|
"num_head_channels": -1,
|
|
}
|
|
|
|
required_keys = {
|
|
"cc_projection.weight": None,
|
|
"cc_projection.bias": None,
|
|
}
|
|
|
|
clip_vision_prefix = "cond_stage_model.model.visual."
|
|
|
|
latent_format = latent_formats.SD15
|
|
|
|
def get_model(self, state_dict, prefix="", device=None):
|
|
out = model_base.Stable_Zero123(self, device=device, cc_projection_weight=state_dict["cc_projection.weight"], cc_projection_bias=state_dict["cc_projection.bias"])
|
|
return out
|
|
|
|
def clip_target(self):
|
|
return None
|
|
|
|
class SD_X4Upscaler(SD20):
|
|
unet_config = {
|
|
"context_dim": 1024,
|
|
"model_channels": 256,
|
|
'in_channels': 7,
|
|
"use_linear_in_transformer": True,
|
|
"adm_in_channels": None,
|
|
"use_temporal_attention": False,
|
|
}
|
|
|
|
unet_extra_config = {
|
|
"disable_self_attentions": [True, True, True, False],
|
|
"num_classes": 1000,
|
|
"num_heads": 8,
|
|
"num_head_channels": -1,
|
|
}
|
|
|
|
latent_format = latent_formats.SD_X4
|
|
|
|
sampling_settings = {
|
|
"linear_start": 0.0001,
|
|
"linear_end": 0.02,
|
|
}
|
|
|
|
def get_model(self, state_dict, prefix="", device=None):
|
|
out = model_base.SD_X4Upscaler(self, device=device)
|
|
return out
|
|
|
|
class Stable_Cascade_C(supported_models_base.BASE):
|
|
unet_config = {
|
|
"stable_cascade_stage": 'c',
|
|
}
|
|
|
|
unet_extra_config = {}
|
|
|
|
latent_format = latent_formats.SC_Prior
|
|
supported_inference_dtypes = [torch.bfloat16, torch.float32]
|
|
|
|
sampling_settings = {
|
|
"shift": 2.0,
|
|
}
|
|
|
|
vae_key_prefix = ["vae."]
|
|
text_encoder_key_prefix = ["text_encoder."]
|
|
clip_vision_prefix = "clip_l_vision."
|
|
|
|
def process_unet_state_dict(self, state_dict):
|
|
key_list = list(state_dict.keys())
|
|
for y in ["weight", "bias"]:
|
|
suffix = "in_proj_{}".format(y)
|
|
keys = filter(lambda a: a.endswith(suffix), key_list)
|
|
for k_from in keys:
|
|
weights = state_dict.pop(k_from)
|
|
prefix = k_from[:-(len(suffix) + 1)]
|
|
shape_from = weights.shape[0] // 3
|
|
for x in range(3):
|
|
p = ["to_q", "to_k", "to_v"]
|
|
k_to = "{}.{}.{}".format(prefix, p[x], y)
|
|
state_dict[k_to] = weights[shape_from*x:shape_from*(x + 1)]
|
|
return state_dict
|
|
|
|
def process_clip_state_dict(self, state_dict):
|
|
state_dict = utils.state_dict_prefix_replace(state_dict, {k: "" for k in self.text_encoder_key_prefix}, filter_keys=True)
|
|
if "clip_g.text_projection" in state_dict:
|
|
state_dict["clip_g.transformer.text_projection.weight"] = state_dict.pop("clip_g.text_projection").transpose(0, 1)
|
|
return state_dict
|
|
|
|
def get_model(self, state_dict, prefix="", device=None):
|
|
out = model_base.StableCascade_C(self, device=device)
|
|
return out
|
|
|
|
def clip_target(self):
|
|
return supported_models_base.ClipTarget(sdxl_clip.StableCascadeTokenizer, sdxl_clip.StableCascadeClipModel)
|
|
|
|
class Stable_Cascade_B(Stable_Cascade_C):
|
|
unet_config = {
|
|
"stable_cascade_stage": 'b',
|
|
}
|
|
|
|
unet_extra_config = {}
|
|
|
|
latent_format = latent_formats.SC_B
|
|
supported_inference_dtypes = [torch.float16, torch.bfloat16, torch.float32]
|
|
|
|
sampling_settings = {
|
|
"shift": 1.0,
|
|
}
|
|
|
|
clip_vision_prefix = None
|
|
|
|
def get_model(self, state_dict, prefix="", device=None):
|
|
out = model_base.StableCascade_B(self, device=device)
|
|
return out
|
|
|
|
class SD15_instructpix2pix(SD15):
|
|
unet_config = {
|
|
"context_dim": 768,
|
|
"model_channels": 320,
|
|
"use_linear_in_transformer": False,
|
|
"adm_in_channels": None,
|
|
"use_temporal_attention": False,
|
|
"in_channels": 8,
|
|
}
|
|
|
|
def get_model(self, state_dict, prefix="", device=None):
|
|
return model_base.SD15_instructpix2pix(self, device=device)
|
|
|
|
class SDXL_instructpix2pix(SDXL):
|
|
unet_config = {
|
|
"model_channels": 320,
|
|
"use_linear_in_transformer": True,
|
|
"transformer_depth": [0, 0, 2, 2, 10, 10],
|
|
"context_dim": 2048,
|
|
"adm_in_channels": 2816,
|
|
"use_temporal_attention": False,
|
|
"in_channels": 8,
|
|
}
|
|
|
|
def get_model(self, state_dict, prefix="", device=None):
|
|
return model_base.SDXL_instructpix2pix(self, device=device)
|
|
|
|
models = [Stable_Zero123, SD15_instructpix2pix, SD15, SD20, SD21UnclipL, SD21UnclipH, SDXL_instructpix2pix, SDXLRefiner, SDXL, SSD1B, KOALA_700M, KOALA_1B, Segmind_Vega, SD_X4Upscaler, Stable_Cascade_C, Stable_Cascade_B, SV3D_u, SV3D_p]
|
|
|
|
models += [SVD_img2vid]
|